Does a uniform distribution of many p-values give statistical evidence that H0 is true?
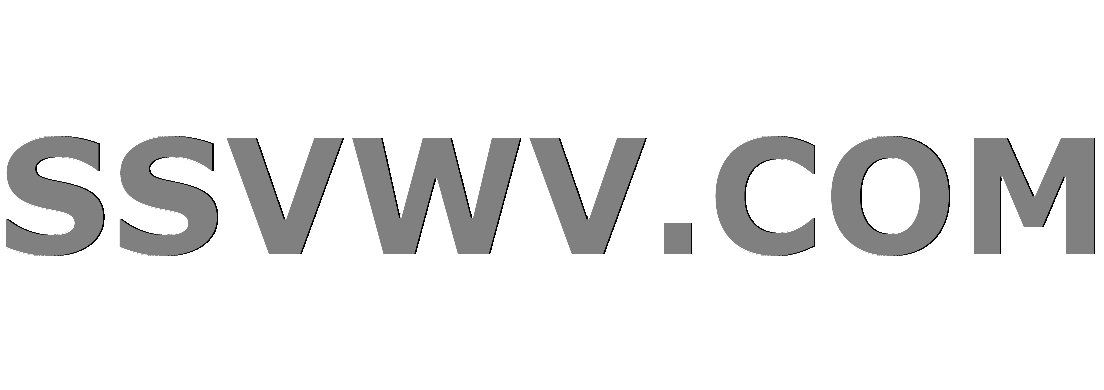
Multi tool use
.everyoneloves__top-leaderboard:empty,.everyoneloves__mid-leaderboard:empty{ margin-bottom:0;
}
up vote
28
down vote
favorite
A single statistical test can give evidence that the null hypothesis (H0) is false and therefore the alternative hypothesis (H1) is true. But it cannot be used to show that H0 is true because failure to reject H0 does not mean that H0 is true.
But let's assume you have the possibility to do the statistical test many times because you have many datasets, all independent of each other. All datasets are the result of the same process and you want to make some statement (H0/H1) over the process itself and aren't interested in the results of each single test. You then collect all the resulting p-values and happen to see via histogram plot that the p-values are clearly uniformly distributed.
My reasoning now is that this can only happen if H0 is true — else the p-values would be distributed differently. Is this therefore enough evidence to conclude that H0 is true? Or am i missing here something essential, because it took me a lot of willpower to write "conclude that H0 is true" which just sounds horribly wrong in my head.
hypothesis-testing p-value combining-p-values
add a comment |
up vote
28
down vote
favorite
A single statistical test can give evidence that the null hypothesis (H0) is false and therefore the alternative hypothesis (H1) is true. But it cannot be used to show that H0 is true because failure to reject H0 does not mean that H0 is true.
But let's assume you have the possibility to do the statistical test many times because you have many datasets, all independent of each other. All datasets are the result of the same process and you want to make some statement (H0/H1) over the process itself and aren't interested in the results of each single test. You then collect all the resulting p-values and happen to see via histogram plot that the p-values are clearly uniformly distributed.
My reasoning now is that this can only happen if H0 is true — else the p-values would be distributed differently. Is this therefore enough evidence to conclude that H0 is true? Or am i missing here something essential, because it took me a lot of willpower to write "conclude that H0 is true" which just sounds horribly wrong in my head.
hypothesis-testing p-value combining-p-values
1
You might be interested in my answer to a different question stats.stackexchange.com/questions/171742/… which has some comments about the hypotheses here.
– mdewey
Nov 13 at 15:59
H0 is false by its definition.
– Joshua
Nov 13 at 17:18
1
On a side note, the reason why i have so many tests (and haven't just combined all the data into a single one) is that my data is spatially distributed around the globe and i wanted to see whether there are spatial patterns in the p-values (there aren't, but if there were it would mean that either independence is violated or that H0/H1 is true in different parts of the globe). I haven't included this in the question text because i wanted to keep it general.
– Leander Moesinger
Nov 13 at 18:20
add a comment |
up vote
28
down vote
favorite
up vote
28
down vote
favorite
A single statistical test can give evidence that the null hypothesis (H0) is false and therefore the alternative hypothesis (H1) is true. But it cannot be used to show that H0 is true because failure to reject H0 does not mean that H0 is true.
But let's assume you have the possibility to do the statistical test many times because you have many datasets, all independent of each other. All datasets are the result of the same process and you want to make some statement (H0/H1) over the process itself and aren't interested in the results of each single test. You then collect all the resulting p-values and happen to see via histogram plot that the p-values are clearly uniformly distributed.
My reasoning now is that this can only happen if H0 is true — else the p-values would be distributed differently. Is this therefore enough evidence to conclude that H0 is true? Or am i missing here something essential, because it took me a lot of willpower to write "conclude that H0 is true" which just sounds horribly wrong in my head.
hypothesis-testing p-value combining-p-values
A single statistical test can give evidence that the null hypothesis (H0) is false and therefore the alternative hypothesis (H1) is true. But it cannot be used to show that H0 is true because failure to reject H0 does not mean that H0 is true.
But let's assume you have the possibility to do the statistical test many times because you have many datasets, all independent of each other. All datasets are the result of the same process and you want to make some statement (H0/H1) over the process itself and aren't interested in the results of each single test. You then collect all the resulting p-values and happen to see via histogram plot that the p-values are clearly uniformly distributed.
My reasoning now is that this can only happen if H0 is true — else the p-values would be distributed differently. Is this therefore enough evidence to conclude that H0 is true? Or am i missing here something essential, because it took me a lot of willpower to write "conclude that H0 is true" which just sounds horribly wrong in my head.
hypothesis-testing p-value combining-p-values
hypothesis-testing p-value combining-p-values
edited Nov 14 at 14:35
mdewey
11.4k72041
11.4k72041
asked Nov 13 at 15:22
Leander Moesinger
27429
27429
1
You might be interested in my answer to a different question stats.stackexchange.com/questions/171742/… which has some comments about the hypotheses here.
– mdewey
Nov 13 at 15:59
H0 is false by its definition.
– Joshua
Nov 13 at 17:18
1
On a side note, the reason why i have so many tests (and haven't just combined all the data into a single one) is that my data is spatially distributed around the globe and i wanted to see whether there are spatial patterns in the p-values (there aren't, but if there were it would mean that either independence is violated or that H0/H1 is true in different parts of the globe). I haven't included this in the question text because i wanted to keep it general.
– Leander Moesinger
Nov 13 at 18:20
add a comment |
1
You might be interested in my answer to a different question stats.stackexchange.com/questions/171742/… which has some comments about the hypotheses here.
– mdewey
Nov 13 at 15:59
H0 is false by its definition.
– Joshua
Nov 13 at 17:18
1
On a side note, the reason why i have so many tests (and haven't just combined all the data into a single one) is that my data is spatially distributed around the globe and i wanted to see whether there are spatial patterns in the p-values (there aren't, but if there were it would mean that either independence is violated or that H0/H1 is true in different parts of the globe). I haven't included this in the question text because i wanted to keep it general.
– Leander Moesinger
Nov 13 at 18:20
1
1
You might be interested in my answer to a different question stats.stackexchange.com/questions/171742/… which has some comments about the hypotheses here.
– mdewey
Nov 13 at 15:59
You might be interested in my answer to a different question stats.stackexchange.com/questions/171742/… which has some comments about the hypotheses here.
– mdewey
Nov 13 at 15:59
H0 is false by its definition.
– Joshua
Nov 13 at 17:18
H0 is false by its definition.
– Joshua
Nov 13 at 17:18
1
1
On a side note, the reason why i have so many tests (and haven't just combined all the data into a single one) is that my data is spatially distributed around the globe and i wanted to see whether there are spatial patterns in the p-values (there aren't, but if there were it would mean that either independence is violated or that H0/H1 is true in different parts of the globe). I haven't included this in the question text because i wanted to keep it general.
– Leander Moesinger
Nov 13 at 18:20
On a side note, the reason why i have so many tests (and haven't just combined all the data into a single one) is that my data is spatially distributed around the globe and i wanted to see whether there are spatial patterns in the p-values (there aren't, but if there were it would mean that either independence is violated or that H0/H1 is true in different parts of the globe). I haven't included this in the question text because i wanted to keep it general.
– Leander Moesinger
Nov 13 at 18:20
add a comment |
4 Answers
4
active
oldest
votes
up vote
22
down vote
accepted
I like your question, but unfortunately my answer is NO, it doesn't prove $H_0$. The reason is very simple. How would do you know that the distribution of p-values is uniform? You would probably have to run a test for uniformity which will return you its own p-value, and you end up with the same kind of inference question that you were trying to avoid, only one step farther. Instead of looking at p-value of the original $H_0$, now you look at a p-value of another $H'_0$ about the uniformity of distribution of original p-values.
UPDATE
Here's the demonstration. I generate 100 samples of 100 observations from Gaussian and Poisson distribution, then obtain 100 p-values for normality test of each sample. So, the premise of the question is that if the p-values are from uniform distribution, then it proves that the null hypothesis is correct, which is a stronger statement than a usual "fails to reject" in statistical inference. The trouble is that "the p-values are from uniform" is a hypothesis itself, which you have to somehow test.
In the picture (first row) below I'm showing the histograms of p-values from a normality test for the Guassian and Poisson sample, and you can see that it's hard to say whether one is more uniform than the other. That was my main point.
The second row shows one of the samples from each distribution. The samples are relatively small, so you can't have too many bins indeed. Actually, this particular Gaussian sample doesn't look that much Gaussian at all on the histogram.
In the third row, I'm showing the combined samples of 10,000 observations for each distribution on a histogram. Here, you can have more bins, and the shapes are more obvious.
Finally, I run the same normality test and get p-values for the combined samples and it rejects normality for Poisson, while failing to reject for Gaussian. The p-values are: [0.45348631] [0.]
This is not a proof, of course, but the demonstration of the idea that you better run the same test on the combined sample, instead of trying to analyze the distribution of p-values from subsamples.
Here's Python code:
import numpy as np
from scipy import stats
from matplotlib import pyplot as plt
def pvs(x):
pn = x.shape[1]
pvals = np.zeros(pn)
for i in range(pn):
pvals[i] = stats.jarque_bera(x[:,i])[1]
return pvals
n = 100
pn = 100
mu, sigma = 1, 2
np.random.seed(0)
x = np.random.normal(mu, sigma, size=(n,pn))
x2 = np.random.poisson(15, size=(n,pn))
print(x[1,1])
pvals = pvs(x)
pvals2 = pvs(x2)
x_f = x.reshape((n*pn,1))
pvals_f = pvs(x_f)
x2_f = x2.reshape((n*pn,1))
pvals2_f = pvs(x2_f)
print(pvals_f,pvals2_f)
print(x_f.shape,x_f[:,0])
#print(pvals)
plt.figure(figsize=(9,9))
plt.subplot(3,2,1)
plt.hist(pvals)
plt.gca().set_title('True Normal')
plt.gca().set_ylabel('p-value')
plt.subplot(3,2,2)
plt.hist(pvals2)
plt.gca().set_title('Poisson')
plt.gca().set_ylabel('p-value')
plt.subplot(3,2,3)
plt.hist(x[:,0])
plt.gca().set_title('a small sample')
plt.gca().set_ylabel('x')
plt.subplot(3,2,4)
plt.hist(x2[:,0])
plt.gca().set_title('a small Sample')
plt.gca().set_ylabel('x')
plt.subplot(3,2,5)
plt.hist(x_f[:,0],100)
plt.gca().set_title('Full Sample')
plt.gca().set_ylabel('x')
plt.subplot(3,2,6)
plt.hist(x2_f[:,0],100)
plt.gca().set_title('Full Sample')
plt.gca().set_ylabel('x')
plt.show()
2
@LeanderMoesinger you're going to make a stronger point by collecting all your tests into one. Suppose, you have a sample with 100 observations, and get p-value; then get 99 additional samples and end up with 100 p-values. Instead, you could just run one 10,000 observations sample and get on p-value, but it'll be more convincing.
– Aksakal
Nov 13 at 17:15
1
@LeanderMoesinger, it's likely to be not small
– Aksakal
Nov 13 at 17:18
1
Your answer does not address the question, he didn’t ask about proof but about evidence.
– Carlos Cinelli
Nov 13 at 18:46
3
@CarlosCinelli, he'll have a bunch of p-values, which he would claim are uniform. How is this an evidence unless he proves the values are from uniform? That's what I'm talking about.
– Aksakal
Nov 13 at 19:21
2
@Aksakal this is about mathematics, an observed event (like a sequence of p-values) may not constitute evidence of something, but the reason does not logically follow from your argument.
– Carlos Cinelli
Nov 13 at 20:52
|
show 5 more comments
up vote
21
down vote
Your series of experiments can be viewed as a single experiment with far more data, and as we know, more data is advantageous (eg. typically standard errors decrease as $sqrt{n}$ increases for independent data). But you ask, "Is this ... enough evidence to conclude that H0 is true?"
No. A basic problem is that another theory may produce similar patterns in data! As @Björn discusses in his answer, you will fail to reject a false $H_0$ if your experiment lacks power to distinguish $H_0$ from other possibilities. For centuries, we failed to reject Newton's theory of gravity because no one had conducted the types of tests where Newton's theory gives sufficiently different predictions than Einstein's theory of general relativity. Less extreme examples are commonplace.
David Hume and the problem of induction
Perhaps a rephrasing is, "If I obtain more and more data consistent with $H_0$ being true, can I ever conclude that $H_0$ is true?"
That question is deeply related to 18th century philosopher David Hume's problem of induction. If all observed instances of A have been B, can we say that the next instance of A will be B? Hume famously said no, that we cannot logically deduce that "all A are B" even from voluminous data. In more modern math, a finite set of observations cannot logically entail $forall_{a in A} left[ a in B right]$ if A is not a finite set. Two notable examples as discussed by Magee and Passermore:
For centuries, every swan observed by Europeans was white. Then Europeans discovered Australia and saw black swans.
For centuries, Newton's law of gravity agreed with observation and was thought correct. It was overturned though by Einstein's theory of general relativity.
If Hume's conclusion is correct, proving $H_0$ true is unachievable. That we cannot make statements with certitude though is not equivalent to saying we know nothing at all. Experimental science and statistics have been successful in helping us understand and navigate the world.
An (incomplete) listing of ways forward:
Karl Popper and falsificationism
In Karl Popper's view, no scientific law is ever proven true. We only have scientific laws not yet proven false.
Popper argued that science proceeds forward by guessing hypotheses and subjecting them to rigorous scrutiny. It proceeds forward through deduction (observation proving theories false), not induction (repeated observation proving theories true). Much of frequentist statistics was constructed consistent with this philosophy.
Popper's view has been immensely influential, but as Kuhn and others have argued, it does not quite conform to the empirically observed practice of successful science.
Bayesian, subjective probability
Let's assume we're interested in a parameter $theta$.
To the frequentist statistician, parameter $theta$ is a scalar value, a number. If you instead take a subjective Bayesian viewpoint (such as in Leonard Jimmie Savage's Foundation of Statistics), you can model your own uncertainty over $theta$ using the tools of probability. To the subjective Bayesian, $theta$ is a random variable and you have some prior $P(theta)$. You can then talk about the subjective probability $P(theta mid X)$ of different values of $theta$ given the data $X$. How you behave in various situations has some correspondence to these subjective probabilities.
This is a logical way to model your own subjective beliefs, but it's not a magic way to produce probabilities that are true in terms of correspondence to reality. A tricky question for any Bayesian interpretation is where do priors come from? Also, what if the model is misspecified?
George P. Box
A famous aphorism of George E.P. Box is that "all models are false, but some are useful."
Newton's law may not be true, but it's still useful for many problems. Box's view is quite important in the modern big data context where studies are so overpowered that you can reject basically any meaningful proposition. Strictly true versus false is a bad question: what matters is whether a model helps you understand the data.
Additional comments
There's a world of difference in statistics between estimating a parameter $theta approx 0$ with a small standard error versus with a large standard error! Don't walk away thinking that because certitude is impossible, passing rigorous scrutiny is irrelevant.
Perhaps also of interest, statistically analyzing the results of multiple studies is called meta-analysis.
How far you can go beyond narrow statistical interpretations is a difficult question.
This has been an interesting read and gave some nice things to think about! I wish i could accept multiple answers.
– Leander Moesinger
Nov 13 at 18:29
Quite an explanation. My prof once summarized Kuhn in the spirit of Popper: 'Science progresses from funeral to funeral'
– skrubber
Nov 13 at 20:24
Kuhn etc famously misinterpret Popper when claiming his observations don't match how science is done. This is known as native falsificationism, and it's not what Popper (later) put forward. It's a straw man.
– Konrad Rudolph
Nov 13 at 22:04
2
It's answers like this I keep visiting StackExchange sites.
– Trilarion
Nov 14 at 16:58
add a comment |
up vote
4
down vote
In a sense you are right (see the p-curve) with some small caveats:
- you need the test to have some power under the alternative. Illustration of the potential problem: generating a p-value as a uniform distribution on 0 to 1 and rejecting when $p leq alpha$ is a (admittedly pretty useless) level $alpha$ test for any null hypothesis, but you will get a uniform distribution of p-values whether $H_0$ is true or not.
- You can only really show that you are quite close to $H_0$ being true (i.e. under the true parameter values three distribution might be close to uniform, even if $H_0$ is false.
With realistic applications, you tend to get additional issues. These mostly arise, because no one person/lab/study group can usually do all the necessary studies. As a result one tends to look at studies from lots of groups, at which point you have increased concerns (i.e. if you had done all relevant experiments yourself, at least you'd know) of underreporting, selective reporting of significant/surprising findings, p-hacking, multiple testing/multiple testing corrections and so on.
(+1) The power point is hugely important! Different theories can produce observationally equivalent data, and a critical part of experiment design is to produce and/or collect data that would allow you to distinguish.
– Matthew Gunn
Nov 15 at 16:35
add a comment |
up vote
-2
down vote
Null hypothesis (H0): Gravity causes everything in the universe to fall toward Earth's surface.
Alternate hypothesis (H1): Nothing ever falls.
Performed 1 million experiments with dozens of household objects, fail to reject H0 with $p < 0.01$ every time. Is H0 true?
New contributor
usul is a new contributor to this site. Take care in asking for clarification, commenting, and answering.
Check out our Code of Conduct.
2
Do you think Galileo did one million trials? None of this stuff is necessary in physical sciences. Establishing the laws of nature by applying scientific method does not reduce into statistical inference.
– Aksakal
Nov 13 at 20:11
1
-1 This is scientifically, statistically, and historically inaccurate. Greeks once believed that it was affinity that drew objects to the Earth. Not bad, but doesn't explain the 3+ body system problems well. Hypotheses should be complementary. Lastly stating a possibly known bias as H_0 and showing experiments continue to lead to the same incorrect conclusion doesn't make the conclusion correct. e.g. women earn less than men b/c they are less driven, sample all women's salaries, H_0 is true!
– AdamO
Nov 13 at 21:00
@AdamO that is exactly my point.
– usul
Nov 13 at 21:01
@AdamO, in the Western countries women earn less when they work less for a variety of reasons including their own choice, disincentives of all kinds and hostile work environment in some places. When they work the same, they earn about the same, e.g. see medicare nurse salaries where women are the great majority: medscape.com/slideshow/…. They all earn the same $37 when working hourly. Totally off-topic, of course.
– Aksakal
Nov 13 at 21:27
2
If your null hypothesis isGravity causes everything in the universe to fall toward Earth's surface
isn't the alternative hypothesisThere is at least one thing in the universe that does not fall toward the Earth's surface
and notNothing ever falls
?
– Eff
Nov 14 at 8:13
|
show 2 more comments
4 Answers
4
active
oldest
votes
4 Answers
4
active
oldest
votes
active
oldest
votes
active
oldest
votes
up vote
22
down vote
accepted
I like your question, but unfortunately my answer is NO, it doesn't prove $H_0$. The reason is very simple. How would do you know that the distribution of p-values is uniform? You would probably have to run a test for uniformity which will return you its own p-value, and you end up with the same kind of inference question that you were trying to avoid, only one step farther. Instead of looking at p-value of the original $H_0$, now you look at a p-value of another $H'_0$ about the uniformity of distribution of original p-values.
UPDATE
Here's the demonstration. I generate 100 samples of 100 observations from Gaussian and Poisson distribution, then obtain 100 p-values for normality test of each sample. So, the premise of the question is that if the p-values are from uniform distribution, then it proves that the null hypothesis is correct, which is a stronger statement than a usual "fails to reject" in statistical inference. The trouble is that "the p-values are from uniform" is a hypothesis itself, which you have to somehow test.
In the picture (first row) below I'm showing the histograms of p-values from a normality test for the Guassian and Poisson sample, and you can see that it's hard to say whether one is more uniform than the other. That was my main point.
The second row shows one of the samples from each distribution. The samples are relatively small, so you can't have too many bins indeed. Actually, this particular Gaussian sample doesn't look that much Gaussian at all on the histogram.
In the third row, I'm showing the combined samples of 10,000 observations for each distribution on a histogram. Here, you can have more bins, and the shapes are more obvious.
Finally, I run the same normality test and get p-values for the combined samples and it rejects normality for Poisson, while failing to reject for Gaussian. The p-values are: [0.45348631] [0.]
This is not a proof, of course, but the demonstration of the idea that you better run the same test on the combined sample, instead of trying to analyze the distribution of p-values from subsamples.
Here's Python code:
import numpy as np
from scipy import stats
from matplotlib import pyplot as plt
def pvs(x):
pn = x.shape[1]
pvals = np.zeros(pn)
for i in range(pn):
pvals[i] = stats.jarque_bera(x[:,i])[1]
return pvals
n = 100
pn = 100
mu, sigma = 1, 2
np.random.seed(0)
x = np.random.normal(mu, sigma, size=(n,pn))
x2 = np.random.poisson(15, size=(n,pn))
print(x[1,1])
pvals = pvs(x)
pvals2 = pvs(x2)
x_f = x.reshape((n*pn,1))
pvals_f = pvs(x_f)
x2_f = x2.reshape((n*pn,1))
pvals2_f = pvs(x2_f)
print(pvals_f,pvals2_f)
print(x_f.shape,x_f[:,0])
#print(pvals)
plt.figure(figsize=(9,9))
plt.subplot(3,2,1)
plt.hist(pvals)
plt.gca().set_title('True Normal')
plt.gca().set_ylabel('p-value')
plt.subplot(3,2,2)
plt.hist(pvals2)
plt.gca().set_title('Poisson')
plt.gca().set_ylabel('p-value')
plt.subplot(3,2,3)
plt.hist(x[:,0])
plt.gca().set_title('a small sample')
plt.gca().set_ylabel('x')
plt.subplot(3,2,4)
plt.hist(x2[:,0])
plt.gca().set_title('a small Sample')
plt.gca().set_ylabel('x')
plt.subplot(3,2,5)
plt.hist(x_f[:,0],100)
plt.gca().set_title('Full Sample')
plt.gca().set_ylabel('x')
plt.subplot(3,2,6)
plt.hist(x2_f[:,0],100)
plt.gca().set_title('Full Sample')
plt.gca().set_ylabel('x')
plt.show()
2
@LeanderMoesinger you're going to make a stronger point by collecting all your tests into one. Suppose, you have a sample with 100 observations, and get p-value; then get 99 additional samples and end up with 100 p-values. Instead, you could just run one 10,000 observations sample and get on p-value, but it'll be more convincing.
– Aksakal
Nov 13 at 17:15
1
@LeanderMoesinger, it's likely to be not small
– Aksakal
Nov 13 at 17:18
1
Your answer does not address the question, he didn’t ask about proof but about evidence.
– Carlos Cinelli
Nov 13 at 18:46
3
@CarlosCinelli, he'll have a bunch of p-values, which he would claim are uniform. How is this an evidence unless he proves the values are from uniform? That's what I'm talking about.
– Aksakal
Nov 13 at 19:21
2
@Aksakal this is about mathematics, an observed event (like a sequence of p-values) may not constitute evidence of something, but the reason does not logically follow from your argument.
– Carlos Cinelli
Nov 13 at 20:52
|
show 5 more comments
up vote
22
down vote
accepted
I like your question, but unfortunately my answer is NO, it doesn't prove $H_0$. The reason is very simple. How would do you know that the distribution of p-values is uniform? You would probably have to run a test for uniformity which will return you its own p-value, and you end up with the same kind of inference question that you were trying to avoid, only one step farther. Instead of looking at p-value of the original $H_0$, now you look at a p-value of another $H'_0$ about the uniformity of distribution of original p-values.
UPDATE
Here's the demonstration. I generate 100 samples of 100 observations from Gaussian and Poisson distribution, then obtain 100 p-values for normality test of each sample. So, the premise of the question is that if the p-values are from uniform distribution, then it proves that the null hypothesis is correct, which is a stronger statement than a usual "fails to reject" in statistical inference. The trouble is that "the p-values are from uniform" is a hypothesis itself, which you have to somehow test.
In the picture (first row) below I'm showing the histograms of p-values from a normality test for the Guassian and Poisson sample, and you can see that it's hard to say whether one is more uniform than the other. That was my main point.
The second row shows one of the samples from each distribution. The samples are relatively small, so you can't have too many bins indeed. Actually, this particular Gaussian sample doesn't look that much Gaussian at all on the histogram.
In the third row, I'm showing the combined samples of 10,000 observations for each distribution on a histogram. Here, you can have more bins, and the shapes are more obvious.
Finally, I run the same normality test and get p-values for the combined samples and it rejects normality for Poisson, while failing to reject for Gaussian. The p-values are: [0.45348631] [0.]
This is not a proof, of course, but the demonstration of the idea that you better run the same test on the combined sample, instead of trying to analyze the distribution of p-values from subsamples.
Here's Python code:
import numpy as np
from scipy import stats
from matplotlib import pyplot as plt
def pvs(x):
pn = x.shape[1]
pvals = np.zeros(pn)
for i in range(pn):
pvals[i] = stats.jarque_bera(x[:,i])[1]
return pvals
n = 100
pn = 100
mu, sigma = 1, 2
np.random.seed(0)
x = np.random.normal(mu, sigma, size=(n,pn))
x2 = np.random.poisson(15, size=(n,pn))
print(x[1,1])
pvals = pvs(x)
pvals2 = pvs(x2)
x_f = x.reshape((n*pn,1))
pvals_f = pvs(x_f)
x2_f = x2.reshape((n*pn,1))
pvals2_f = pvs(x2_f)
print(pvals_f,pvals2_f)
print(x_f.shape,x_f[:,0])
#print(pvals)
plt.figure(figsize=(9,9))
plt.subplot(3,2,1)
plt.hist(pvals)
plt.gca().set_title('True Normal')
plt.gca().set_ylabel('p-value')
plt.subplot(3,2,2)
plt.hist(pvals2)
plt.gca().set_title('Poisson')
plt.gca().set_ylabel('p-value')
plt.subplot(3,2,3)
plt.hist(x[:,0])
plt.gca().set_title('a small sample')
plt.gca().set_ylabel('x')
plt.subplot(3,2,4)
plt.hist(x2[:,0])
plt.gca().set_title('a small Sample')
plt.gca().set_ylabel('x')
plt.subplot(3,2,5)
plt.hist(x_f[:,0],100)
plt.gca().set_title('Full Sample')
plt.gca().set_ylabel('x')
plt.subplot(3,2,6)
plt.hist(x2_f[:,0],100)
plt.gca().set_title('Full Sample')
plt.gca().set_ylabel('x')
plt.show()
2
@LeanderMoesinger you're going to make a stronger point by collecting all your tests into one. Suppose, you have a sample with 100 observations, and get p-value; then get 99 additional samples and end up with 100 p-values. Instead, you could just run one 10,000 observations sample and get on p-value, but it'll be more convincing.
– Aksakal
Nov 13 at 17:15
1
@LeanderMoesinger, it's likely to be not small
– Aksakal
Nov 13 at 17:18
1
Your answer does not address the question, he didn’t ask about proof but about evidence.
– Carlos Cinelli
Nov 13 at 18:46
3
@CarlosCinelli, he'll have a bunch of p-values, which he would claim are uniform. How is this an evidence unless he proves the values are from uniform? That's what I'm talking about.
– Aksakal
Nov 13 at 19:21
2
@Aksakal this is about mathematics, an observed event (like a sequence of p-values) may not constitute evidence of something, but the reason does not logically follow from your argument.
– Carlos Cinelli
Nov 13 at 20:52
|
show 5 more comments
up vote
22
down vote
accepted
up vote
22
down vote
accepted
I like your question, but unfortunately my answer is NO, it doesn't prove $H_0$. The reason is very simple. How would do you know that the distribution of p-values is uniform? You would probably have to run a test for uniformity which will return you its own p-value, and you end up with the same kind of inference question that you were trying to avoid, only one step farther. Instead of looking at p-value of the original $H_0$, now you look at a p-value of another $H'_0$ about the uniformity of distribution of original p-values.
UPDATE
Here's the demonstration. I generate 100 samples of 100 observations from Gaussian and Poisson distribution, then obtain 100 p-values for normality test of each sample. So, the premise of the question is that if the p-values are from uniform distribution, then it proves that the null hypothesis is correct, which is a stronger statement than a usual "fails to reject" in statistical inference. The trouble is that "the p-values are from uniform" is a hypothesis itself, which you have to somehow test.
In the picture (first row) below I'm showing the histograms of p-values from a normality test for the Guassian and Poisson sample, and you can see that it's hard to say whether one is more uniform than the other. That was my main point.
The second row shows one of the samples from each distribution. The samples are relatively small, so you can't have too many bins indeed. Actually, this particular Gaussian sample doesn't look that much Gaussian at all on the histogram.
In the third row, I'm showing the combined samples of 10,000 observations for each distribution on a histogram. Here, you can have more bins, and the shapes are more obvious.
Finally, I run the same normality test and get p-values for the combined samples and it rejects normality for Poisson, while failing to reject for Gaussian. The p-values are: [0.45348631] [0.]
This is not a proof, of course, but the demonstration of the idea that you better run the same test on the combined sample, instead of trying to analyze the distribution of p-values from subsamples.
Here's Python code:
import numpy as np
from scipy import stats
from matplotlib import pyplot as plt
def pvs(x):
pn = x.shape[1]
pvals = np.zeros(pn)
for i in range(pn):
pvals[i] = stats.jarque_bera(x[:,i])[1]
return pvals
n = 100
pn = 100
mu, sigma = 1, 2
np.random.seed(0)
x = np.random.normal(mu, sigma, size=(n,pn))
x2 = np.random.poisson(15, size=(n,pn))
print(x[1,1])
pvals = pvs(x)
pvals2 = pvs(x2)
x_f = x.reshape((n*pn,1))
pvals_f = pvs(x_f)
x2_f = x2.reshape((n*pn,1))
pvals2_f = pvs(x2_f)
print(pvals_f,pvals2_f)
print(x_f.shape,x_f[:,0])
#print(pvals)
plt.figure(figsize=(9,9))
plt.subplot(3,2,1)
plt.hist(pvals)
plt.gca().set_title('True Normal')
plt.gca().set_ylabel('p-value')
plt.subplot(3,2,2)
plt.hist(pvals2)
plt.gca().set_title('Poisson')
plt.gca().set_ylabel('p-value')
plt.subplot(3,2,3)
plt.hist(x[:,0])
plt.gca().set_title('a small sample')
plt.gca().set_ylabel('x')
plt.subplot(3,2,4)
plt.hist(x2[:,0])
plt.gca().set_title('a small Sample')
plt.gca().set_ylabel('x')
plt.subplot(3,2,5)
plt.hist(x_f[:,0],100)
plt.gca().set_title('Full Sample')
plt.gca().set_ylabel('x')
plt.subplot(3,2,6)
plt.hist(x2_f[:,0],100)
plt.gca().set_title('Full Sample')
plt.gca().set_ylabel('x')
plt.show()
I like your question, but unfortunately my answer is NO, it doesn't prove $H_0$. The reason is very simple. How would do you know that the distribution of p-values is uniform? You would probably have to run a test for uniformity which will return you its own p-value, and you end up with the same kind of inference question that you were trying to avoid, only one step farther. Instead of looking at p-value of the original $H_0$, now you look at a p-value of another $H'_0$ about the uniformity of distribution of original p-values.
UPDATE
Here's the demonstration. I generate 100 samples of 100 observations from Gaussian and Poisson distribution, then obtain 100 p-values for normality test of each sample. So, the premise of the question is that if the p-values are from uniform distribution, then it proves that the null hypothesis is correct, which is a stronger statement than a usual "fails to reject" in statistical inference. The trouble is that "the p-values are from uniform" is a hypothesis itself, which you have to somehow test.
In the picture (first row) below I'm showing the histograms of p-values from a normality test for the Guassian and Poisson sample, and you can see that it's hard to say whether one is more uniform than the other. That was my main point.
The second row shows one of the samples from each distribution. The samples are relatively small, so you can't have too many bins indeed. Actually, this particular Gaussian sample doesn't look that much Gaussian at all on the histogram.
In the third row, I'm showing the combined samples of 10,000 observations for each distribution on a histogram. Here, you can have more bins, and the shapes are more obvious.
Finally, I run the same normality test and get p-values for the combined samples and it rejects normality for Poisson, while failing to reject for Gaussian. The p-values are: [0.45348631] [0.]
This is not a proof, of course, but the demonstration of the idea that you better run the same test on the combined sample, instead of trying to analyze the distribution of p-values from subsamples.
Here's Python code:
import numpy as np
from scipy import stats
from matplotlib import pyplot as plt
def pvs(x):
pn = x.shape[1]
pvals = np.zeros(pn)
for i in range(pn):
pvals[i] = stats.jarque_bera(x[:,i])[1]
return pvals
n = 100
pn = 100
mu, sigma = 1, 2
np.random.seed(0)
x = np.random.normal(mu, sigma, size=(n,pn))
x2 = np.random.poisson(15, size=(n,pn))
print(x[1,1])
pvals = pvs(x)
pvals2 = pvs(x2)
x_f = x.reshape((n*pn,1))
pvals_f = pvs(x_f)
x2_f = x2.reshape((n*pn,1))
pvals2_f = pvs(x2_f)
print(pvals_f,pvals2_f)
print(x_f.shape,x_f[:,0])
#print(pvals)
plt.figure(figsize=(9,9))
plt.subplot(3,2,1)
plt.hist(pvals)
plt.gca().set_title('True Normal')
plt.gca().set_ylabel('p-value')
plt.subplot(3,2,2)
plt.hist(pvals2)
plt.gca().set_title('Poisson')
plt.gca().set_ylabel('p-value')
plt.subplot(3,2,3)
plt.hist(x[:,0])
plt.gca().set_title('a small sample')
plt.gca().set_ylabel('x')
plt.subplot(3,2,4)
plt.hist(x2[:,0])
plt.gca().set_title('a small Sample')
plt.gca().set_ylabel('x')
plt.subplot(3,2,5)
plt.hist(x_f[:,0],100)
plt.gca().set_title('Full Sample')
plt.gca().set_ylabel('x')
plt.subplot(3,2,6)
plt.hist(x2_f[:,0],100)
plt.gca().set_title('Full Sample')
plt.gca().set_ylabel('x')
plt.show()
edited Nov 14 at 18:50
answered Nov 13 at 16:14


Aksakal
37.6k447111
37.6k447111
2
@LeanderMoesinger you're going to make a stronger point by collecting all your tests into one. Suppose, you have a sample with 100 observations, and get p-value; then get 99 additional samples and end up with 100 p-values. Instead, you could just run one 10,000 observations sample and get on p-value, but it'll be more convincing.
– Aksakal
Nov 13 at 17:15
1
@LeanderMoesinger, it's likely to be not small
– Aksakal
Nov 13 at 17:18
1
Your answer does not address the question, he didn’t ask about proof but about evidence.
– Carlos Cinelli
Nov 13 at 18:46
3
@CarlosCinelli, he'll have a bunch of p-values, which he would claim are uniform. How is this an evidence unless he proves the values are from uniform? That's what I'm talking about.
– Aksakal
Nov 13 at 19:21
2
@Aksakal this is about mathematics, an observed event (like a sequence of p-values) may not constitute evidence of something, but the reason does not logically follow from your argument.
– Carlos Cinelli
Nov 13 at 20:52
|
show 5 more comments
2
@LeanderMoesinger you're going to make a stronger point by collecting all your tests into one. Suppose, you have a sample with 100 observations, and get p-value; then get 99 additional samples and end up with 100 p-values. Instead, you could just run one 10,000 observations sample and get on p-value, but it'll be more convincing.
– Aksakal
Nov 13 at 17:15
1
@LeanderMoesinger, it's likely to be not small
– Aksakal
Nov 13 at 17:18
1
Your answer does not address the question, he didn’t ask about proof but about evidence.
– Carlos Cinelli
Nov 13 at 18:46
3
@CarlosCinelli, he'll have a bunch of p-values, which he would claim are uniform. How is this an evidence unless he proves the values are from uniform? That's what I'm talking about.
– Aksakal
Nov 13 at 19:21
2
@Aksakal this is about mathematics, an observed event (like a sequence of p-values) may not constitute evidence of something, but the reason does not logically follow from your argument.
– Carlos Cinelli
Nov 13 at 20:52
2
2
@LeanderMoesinger you're going to make a stronger point by collecting all your tests into one. Suppose, you have a sample with 100 observations, and get p-value; then get 99 additional samples and end up with 100 p-values. Instead, you could just run one 10,000 observations sample and get on p-value, but it'll be more convincing.
– Aksakal
Nov 13 at 17:15
@LeanderMoesinger you're going to make a stronger point by collecting all your tests into one. Suppose, you have a sample with 100 observations, and get p-value; then get 99 additional samples and end up with 100 p-values. Instead, you could just run one 10,000 observations sample and get on p-value, but it'll be more convincing.
– Aksakal
Nov 13 at 17:15
1
1
@LeanderMoesinger, it's likely to be not small
– Aksakal
Nov 13 at 17:18
@LeanderMoesinger, it's likely to be not small
– Aksakal
Nov 13 at 17:18
1
1
Your answer does not address the question, he didn’t ask about proof but about evidence.
– Carlos Cinelli
Nov 13 at 18:46
Your answer does not address the question, he didn’t ask about proof but about evidence.
– Carlos Cinelli
Nov 13 at 18:46
3
3
@CarlosCinelli, he'll have a bunch of p-values, which he would claim are uniform. How is this an evidence unless he proves the values are from uniform? That's what I'm talking about.
– Aksakal
Nov 13 at 19:21
@CarlosCinelli, he'll have a bunch of p-values, which he would claim are uniform. How is this an evidence unless he proves the values are from uniform? That's what I'm talking about.
– Aksakal
Nov 13 at 19:21
2
2
@Aksakal this is about mathematics, an observed event (like a sequence of p-values) may not constitute evidence of something, but the reason does not logically follow from your argument.
– Carlos Cinelli
Nov 13 at 20:52
@Aksakal this is about mathematics, an observed event (like a sequence of p-values) may not constitute evidence of something, but the reason does not logically follow from your argument.
– Carlos Cinelli
Nov 13 at 20:52
|
show 5 more comments
up vote
21
down vote
Your series of experiments can be viewed as a single experiment with far more data, and as we know, more data is advantageous (eg. typically standard errors decrease as $sqrt{n}$ increases for independent data). But you ask, "Is this ... enough evidence to conclude that H0 is true?"
No. A basic problem is that another theory may produce similar patterns in data! As @Björn discusses in his answer, you will fail to reject a false $H_0$ if your experiment lacks power to distinguish $H_0$ from other possibilities. For centuries, we failed to reject Newton's theory of gravity because no one had conducted the types of tests where Newton's theory gives sufficiently different predictions than Einstein's theory of general relativity. Less extreme examples are commonplace.
David Hume and the problem of induction
Perhaps a rephrasing is, "If I obtain more and more data consistent with $H_0$ being true, can I ever conclude that $H_0$ is true?"
That question is deeply related to 18th century philosopher David Hume's problem of induction. If all observed instances of A have been B, can we say that the next instance of A will be B? Hume famously said no, that we cannot logically deduce that "all A are B" even from voluminous data. In more modern math, a finite set of observations cannot logically entail $forall_{a in A} left[ a in B right]$ if A is not a finite set. Two notable examples as discussed by Magee and Passermore:
For centuries, every swan observed by Europeans was white. Then Europeans discovered Australia and saw black swans.
For centuries, Newton's law of gravity agreed with observation and was thought correct. It was overturned though by Einstein's theory of general relativity.
If Hume's conclusion is correct, proving $H_0$ true is unachievable. That we cannot make statements with certitude though is not equivalent to saying we know nothing at all. Experimental science and statistics have been successful in helping us understand and navigate the world.
An (incomplete) listing of ways forward:
Karl Popper and falsificationism
In Karl Popper's view, no scientific law is ever proven true. We only have scientific laws not yet proven false.
Popper argued that science proceeds forward by guessing hypotheses and subjecting them to rigorous scrutiny. It proceeds forward through deduction (observation proving theories false), not induction (repeated observation proving theories true). Much of frequentist statistics was constructed consistent with this philosophy.
Popper's view has been immensely influential, but as Kuhn and others have argued, it does not quite conform to the empirically observed practice of successful science.
Bayesian, subjective probability
Let's assume we're interested in a parameter $theta$.
To the frequentist statistician, parameter $theta$ is a scalar value, a number. If you instead take a subjective Bayesian viewpoint (such as in Leonard Jimmie Savage's Foundation of Statistics), you can model your own uncertainty over $theta$ using the tools of probability. To the subjective Bayesian, $theta$ is a random variable and you have some prior $P(theta)$. You can then talk about the subjective probability $P(theta mid X)$ of different values of $theta$ given the data $X$. How you behave in various situations has some correspondence to these subjective probabilities.
This is a logical way to model your own subjective beliefs, but it's not a magic way to produce probabilities that are true in terms of correspondence to reality. A tricky question for any Bayesian interpretation is where do priors come from? Also, what if the model is misspecified?
George P. Box
A famous aphorism of George E.P. Box is that "all models are false, but some are useful."
Newton's law may not be true, but it's still useful for many problems. Box's view is quite important in the modern big data context where studies are so overpowered that you can reject basically any meaningful proposition. Strictly true versus false is a bad question: what matters is whether a model helps you understand the data.
Additional comments
There's a world of difference in statistics between estimating a parameter $theta approx 0$ with a small standard error versus with a large standard error! Don't walk away thinking that because certitude is impossible, passing rigorous scrutiny is irrelevant.
Perhaps also of interest, statistically analyzing the results of multiple studies is called meta-analysis.
How far you can go beyond narrow statistical interpretations is a difficult question.
This has been an interesting read and gave some nice things to think about! I wish i could accept multiple answers.
– Leander Moesinger
Nov 13 at 18:29
Quite an explanation. My prof once summarized Kuhn in the spirit of Popper: 'Science progresses from funeral to funeral'
– skrubber
Nov 13 at 20:24
Kuhn etc famously misinterpret Popper when claiming his observations don't match how science is done. This is known as native falsificationism, and it's not what Popper (later) put forward. It's a straw man.
– Konrad Rudolph
Nov 13 at 22:04
2
It's answers like this I keep visiting StackExchange sites.
– Trilarion
Nov 14 at 16:58
add a comment |
up vote
21
down vote
Your series of experiments can be viewed as a single experiment with far more data, and as we know, more data is advantageous (eg. typically standard errors decrease as $sqrt{n}$ increases for independent data). But you ask, "Is this ... enough evidence to conclude that H0 is true?"
No. A basic problem is that another theory may produce similar patterns in data! As @Björn discusses in his answer, you will fail to reject a false $H_0$ if your experiment lacks power to distinguish $H_0$ from other possibilities. For centuries, we failed to reject Newton's theory of gravity because no one had conducted the types of tests where Newton's theory gives sufficiently different predictions than Einstein's theory of general relativity. Less extreme examples are commonplace.
David Hume and the problem of induction
Perhaps a rephrasing is, "If I obtain more and more data consistent with $H_0$ being true, can I ever conclude that $H_0$ is true?"
That question is deeply related to 18th century philosopher David Hume's problem of induction. If all observed instances of A have been B, can we say that the next instance of A will be B? Hume famously said no, that we cannot logically deduce that "all A are B" even from voluminous data. In more modern math, a finite set of observations cannot logically entail $forall_{a in A} left[ a in B right]$ if A is not a finite set. Two notable examples as discussed by Magee and Passermore:
For centuries, every swan observed by Europeans was white. Then Europeans discovered Australia and saw black swans.
For centuries, Newton's law of gravity agreed with observation and was thought correct. It was overturned though by Einstein's theory of general relativity.
If Hume's conclusion is correct, proving $H_0$ true is unachievable. That we cannot make statements with certitude though is not equivalent to saying we know nothing at all. Experimental science and statistics have been successful in helping us understand and navigate the world.
An (incomplete) listing of ways forward:
Karl Popper and falsificationism
In Karl Popper's view, no scientific law is ever proven true. We only have scientific laws not yet proven false.
Popper argued that science proceeds forward by guessing hypotheses and subjecting them to rigorous scrutiny. It proceeds forward through deduction (observation proving theories false), not induction (repeated observation proving theories true). Much of frequentist statistics was constructed consistent with this philosophy.
Popper's view has been immensely influential, but as Kuhn and others have argued, it does not quite conform to the empirically observed practice of successful science.
Bayesian, subjective probability
Let's assume we're interested in a parameter $theta$.
To the frequentist statistician, parameter $theta$ is a scalar value, a number. If you instead take a subjective Bayesian viewpoint (such as in Leonard Jimmie Savage's Foundation of Statistics), you can model your own uncertainty over $theta$ using the tools of probability. To the subjective Bayesian, $theta$ is a random variable and you have some prior $P(theta)$. You can then talk about the subjective probability $P(theta mid X)$ of different values of $theta$ given the data $X$. How you behave in various situations has some correspondence to these subjective probabilities.
This is a logical way to model your own subjective beliefs, but it's not a magic way to produce probabilities that are true in terms of correspondence to reality. A tricky question for any Bayesian interpretation is where do priors come from? Also, what if the model is misspecified?
George P. Box
A famous aphorism of George E.P. Box is that "all models are false, but some are useful."
Newton's law may not be true, but it's still useful for many problems. Box's view is quite important in the modern big data context where studies are so overpowered that you can reject basically any meaningful proposition. Strictly true versus false is a bad question: what matters is whether a model helps you understand the data.
Additional comments
There's a world of difference in statistics between estimating a parameter $theta approx 0$ with a small standard error versus with a large standard error! Don't walk away thinking that because certitude is impossible, passing rigorous scrutiny is irrelevant.
Perhaps also of interest, statistically analyzing the results of multiple studies is called meta-analysis.
How far you can go beyond narrow statistical interpretations is a difficult question.
This has been an interesting read and gave some nice things to think about! I wish i could accept multiple answers.
– Leander Moesinger
Nov 13 at 18:29
Quite an explanation. My prof once summarized Kuhn in the spirit of Popper: 'Science progresses from funeral to funeral'
– skrubber
Nov 13 at 20:24
Kuhn etc famously misinterpret Popper when claiming his observations don't match how science is done. This is known as native falsificationism, and it's not what Popper (later) put forward. It's a straw man.
– Konrad Rudolph
Nov 13 at 22:04
2
It's answers like this I keep visiting StackExchange sites.
– Trilarion
Nov 14 at 16:58
add a comment |
up vote
21
down vote
up vote
21
down vote
Your series of experiments can be viewed as a single experiment with far more data, and as we know, more data is advantageous (eg. typically standard errors decrease as $sqrt{n}$ increases for independent data). But you ask, "Is this ... enough evidence to conclude that H0 is true?"
No. A basic problem is that another theory may produce similar patterns in data! As @Björn discusses in his answer, you will fail to reject a false $H_0$ if your experiment lacks power to distinguish $H_0$ from other possibilities. For centuries, we failed to reject Newton's theory of gravity because no one had conducted the types of tests where Newton's theory gives sufficiently different predictions than Einstein's theory of general relativity. Less extreme examples are commonplace.
David Hume and the problem of induction
Perhaps a rephrasing is, "If I obtain more and more data consistent with $H_0$ being true, can I ever conclude that $H_0$ is true?"
That question is deeply related to 18th century philosopher David Hume's problem of induction. If all observed instances of A have been B, can we say that the next instance of A will be B? Hume famously said no, that we cannot logically deduce that "all A are B" even from voluminous data. In more modern math, a finite set of observations cannot logically entail $forall_{a in A} left[ a in B right]$ if A is not a finite set. Two notable examples as discussed by Magee and Passermore:
For centuries, every swan observed by Europeans was white. Then Europeans discovered Australia and saw black swans.
For centuries, Newton's law of gravity agreed with observation and was thought correct. It was overturned though by Einstein's theory of general relativity.
If Hume's conclusion is correct, proving $H_0$ true is unachievable. That we cannot make statements with certitude though is not equivalent to saying we know nothing at all. Experimental science and statistics have been successful in helping us understand and navigate the world.
An (incomplete) listing of ways forward:
Karl Popper and falsificationism
In Karl Popper's view, no scientific law is ever proven true. We only have scientific laws not yet proven false.
Popper argued that science proceeds forward by guessing hypotheses and subjecting them to rigorous scrutiny. It proceeds forward through deduction (observation proving theories false), not induction (repeated observation proving theories true). Much of frequentist statistics was constructed consistent with this philosophy.
Popper's view has been immensely influential, but as Kuhn and others have argued, it does not quite conform to the empirically observed practice of successful science.
Bayesian, subjective probability
Let's assume we're interested in a parameter $theta$.
To the frequentist statistician, parameter $theta$ is a scalar value, a number. If you instead take a subjective Bayesian viewpoint (such as in Leonard Jimmie Savage's Foundation of Statistics), you can model your own uncertainty over $theta$ using the tools of probability. To the subjective Bayesian, $theta$ is a random variable and you have some prior $P(theta)$. You can then talk about the subjective probability $P(theta mid X)$ of different values of $theta$ given the data $X$. How you behave in various situations has some correspondence to these subjective probabilities.
This is a logical way to model your own subjective beliefs, but it's not a magic way to produce probabilities that are true in terms of correspondence to reality. A tricky question for any Bayesian interpretation is where do priors come from? Also, what if the model is misspecified?
George P. Box
A famous aphorism of George E.P. Box is that "all models are false, but some are useful."
Newton's law may not be true, but it's still useful for many problems. Box's view is quite important in the modern big data context where studies are so overpowered that you can reject basically any meaningful proposition. Strictly true versus false is a bad question: what matters is whether a model helps you understand the data.
Additional comments
There's a world of difference in statistics between estimating a parameter $theta approx 0$ with a small standard error versus with a large standard error! Don't walk away thinking that because certitude is impossible, passing rigorous scrutiny is irrelevant.
Perhaps also of interest, statistically analyzing the results of multiple studies is called meta-analysis.
How far you can go beyond narrow statistical interpretations is a difficult question.
Your series of experiments can be viewed as a single experiment with far more data, and as we know, more data is advantageous (eg. typically standard errors decrease as $sqrt{n}$ increases for independent data). But you ask, "Is this ... enough evidence to conclude that H0 is true?"
No. A basic problem is that another theory may produce similar patterns in data! As @Björn discusses in his answer, you will fail to reject a false $H_0$ if your experiment lacks power to distinguish $H_0$ from other possibilities. For centuries, we failed to reject Newton's theory of gravity because no one had conducted the types of tests where Newton's theory gives sufficiently different predictions than Einstein's theory of general relativity. Less extreme examples are commonplace.
David Hume and the problem of induction
Perhaps a rephrasing is, "If I obtain more and more data consistent with $H_0$ being true, can I ever conclude that $H_0$ is true?"
That question is deeply related to 18th century philosopher David Hume's problem of induction. If all observed instances of A have been B, can we say that the next instance of A will be B? Hume famously said no, that we cannot logically deduce that "all A are B" even from voluminous data. In more modern math, a finite set of observations cannot logically entail $forall_{a in A} left[ a in B right]$ if A is not a finite set. Two notable examples as discussed by Magee and Passermore:
For centuries, every swan observed by Europeans was white. Then Europeans discovered Australia and saw black swans.
For centuries, Newton's law of gravity agreed with observation and was thought correct. It was overturned though by Einstein's theory of general relativity.
If Hume's conclusion is correct, proving $H_0$ true is unachievable. That we cannot make statements with certitude though is not equivalent to saying we know nothing at all. Experimental science and statistics have been successful in helping us understand and navigate the world.
An (incomplete) listing of ways forward:
Karl Popper and falsificationism
In Karl Popper's view, no scientific law is ever proven true. We only have scientific laws not yet proven false.
Popper argued that science proceeds forward by guessing hypotheses and subjecting them to rigorous scrutiny. It proceeds forward through deduction (observation proving theories false), not induction (repeated observation proving theories true). Much of frequentist statistics was constructed consistent with this philosophy.
Popper's view has been immensely influential, but as Kuhn and others have argued, it does not quite conform to the empirically observed practice of successful science.
Bayesian, subjective probability
Let's assume we're interested in a parameter $theta$.
To the frequentist statistician, parameter $theta$ is a scalar value, a number. If you instead take a subjective Bayesian viewpoint (such as in Leonard Jimmie Savage's Foundation of Statistics), you can model your own uncertainty over $theta$ using the tools of probability. To the subjective Bayesian, $theta$ is a random variable and you have some prior $P(theta)$. You can then talk about the subjective probability $P(theta mid X)$ of different values of $theta$ given the data $X$. How you behave in various situations has some correspondence to these subjective probabilities.
This is a logical way to model your own subjective beliefs, but it's not a magic way to produce probabilities that are true in terms of correspondence to reality. A tricky question for any Bayesian interpretation is where do priors come from? Also, what if the model is misspecified?
George P. Box
A famous aphorism of George E.P. Box is that "all models are false, but some are useful."
Newton's law may not be true, but it's still useful for many problems. Box's view is quite important in the modern big data context where studies are so overpowered that you can reject basically any meaningful proposition. Strictly true versus false is a bad question: what matters is whether a model helps you understand the data.
Additional comments
There's a world of difference in statistics between estimating a parameter $theta approx 0$ with a small standard error versus with a large standard error! Don't walk away thinking that because certitude is impossible, passing rigorous scrutiny is irrelevant.
Perhaps also of interest, statistically analyzing the results of multiple studies is called meta-analysis.
How far you can go beyond narrow statistical interpretations is a difficult question.
edited Nov 16 at 3:10
answered Nov 13 at 17:47
Matthew Gunn
16.8k13167
16.8k13167
This has been an interesting read and gave some nice things to think about! I wish i could accept multiple answers.
– Leander Moesinger
Nov 13 at 18:29
Quite an explanation. My prof once summarized Kuhn in the spirit of Popper: 'Science progresses from funeral to funeral'
– skrubber
Nov 13 at 20:24
Kuhn etc famously misinterpret Popper when claiming his observations don't match how science is done. This is known as native falsificationism, and it's not what Popper (later) put forward. It's a straw man.
– Konrad Rudolph
Nov 13 at 22:04
2
It's answers like this I keep visiting StackExchange sites.
– Trilarion
Nov 14 at 16:58
add a comment |
This has been an interesting read and gave some nice things to think about! I wish i could accept multiple answers.
– Leander Moesinger
Nov 13 at 18:29
Quite an explanation. My prof once summarized Kuhn in the spirit of Popper: 'Science progresses from funeral to funeral'
– skrubber
Nov 13 at 20:24
Kuhn etc famously misinterpret Popper when claiming his observations don't match how science is done. This is known as native falsificationism, and it's not what Popper (later) put forward. It's a straw man.
– Konrad Rudolph
Nov 13 at 22:04
2
It's answers like this I keep visiting StackExchange sites.
– Trilarion
Nov 14 at 16:58
This has been an interesting read and gave some nice things to think about! I wish i could accept multiple answers.
– Leander Moesinger
Nov 13 at 18:29
This has been an interesting read and gave some nice things to think about! I wish i could accept multiple answers.
– Leander Moesinger
Nov 13 at 18:29
Quite an explanation. My prof once summarized Kuhn in the spirit of Popper: 'Science progresses from funeral to funeral'
– skrubber
Nov 13 at 20:24
Quite an explanation. My prof once summarized Kuhn in the spirit of Popper: 'Science progresses from funeral to funeral'
– skrubber
Nov 13 at 20:24
Kuhn etc famously misinterpret Popper when claiming his observations don't match how science is done. This is known as native falsificationism, and it's not what Popper (later) put forward. It's a straw man.
– Konrad Rudolph
Nov 13 at 22:04
Kuhn etc famously misinterpret Popper when claiming his observations don't match how science is done. This is known as native falsificationism, and it's not what Popper (later) put forward. It's a straw man.
– Konrad Rudolph
Nov 13 at 22:04
2
2
It's answers like this I keep visiting StackExchange sites.
– Trilarion
Nov 14 at 16:58
It's answers like this I keep visiting StackExchange sites.
– Trilarion
Nov 14 at 16:58
add a comment |
up vote
4
down vote
In a sense you are right (see the p-curve) with some small caveats:
- you need the test to have some power under the alternative. Illustration of the potential problem: generating a p-value as a uniform distribution on 0 to 1 and rejecting when $p leq alpha$ is a (admittedly pretty useless) level $alpha$ test for any null hypothesis, but you will get a uniform distribution of p-values whether $H_0$ is true or not.
- You can only really show that you are quite close to $H_0$ being true (i.e. under the true parameter values three distribution might be close to uniform, even if $H_0$ is false.
With realistic applications, you tend to get additional issues. These mostly arise, because no one person/lab/study group can usually do all the necessary studies. As a result one tends to look at studies from lots of groups, at which point you have increased concerns (i.e. if you had done all relevant experiments yourself, at least you'd know) of underreporting, selective reporting of significant/surprising findings, p-hacking, multiple testing/multiple testing corrections and so on.
(+1) The power point is hugely important! Different theories can produce observationally equivalent data, and a critical part of experiment design is to produce and/or collect data that would allow you to distinguish.
– Matthew Gunn
Nov 15 at 16:35
add a comment |
up vote
4
down vote
In a sense you are right (see the p-curve) with some small caveats:
- you need the test to have some power under the alternative. Illustration of the potential problem: generating a p-value as a uniform distribution on 0 to 1 and rejecting when $p leq alpha$ is a (admittedly pretty useless) level $alpha$ test for any null hypothesis, but you will get a uniform distribution of p-values whether $H_0$ is true or not.
- You can only really show that you are quite close to $H_0$ being true (i.e. under the true parameter values three distribution might be close to uniform, even if $H_0$ is false.
With realistic applications, you tend to get additional issues. These mostly arise, because no one person/lab/study group can usually do all the necessary studies. As a result one tends to look at studies from lots of groups, at which point you have increased concerns (i.e. if you had done all relevant experiments yourself, at least you'd know) of underreporting, selective reporting of significant/surprising findings, p-hacking, multiple testing/multiple testing corrections and so on.
(+1) The power point is hugely important! Different theories can produce observationally equivalent data, and a critical part of experiment design is to produce and/or collect data that would allow you to distinguish.
– Matthew Gunn
Nov 15 at 16:35
add a comment |
up vote
4
down vote
up vote
4
down vote
In a sense you are right (see the p-curve) with some small caveats:
- you need the test to have some power under the alternative. Illustration of the potential problem: generating a p-value as a uniform distribution on 0 to 1 and rejecting when $p leq alpha$ is a (admittedly pretty useless) level $alpha$ test for any null hypothesis, but you will get a uniform distribution of p-values whether $H_0$ is true or not.
- You can only really show that you are quite close to $H_0$ being true (i.e. under the true parameter values three distribution might be close to uniform, even if $H_0$ is false.
With realistic applications, you tend to get additional issues. These mostly arise, because no one person/lab/study group can usually do all the necessary studies. As a result one tends to look at studies from lots of groups, at which point you have increased concerns (i.e. if you had done all relevant experiments yourself, at least you'd know) of underreporting, selective reporting of significant/surprising findings, p-hacking, multiple testing/multiple testing corrections and so on.
In a sense you are right (see the p-curve) with some small caveats:
- you need the test to have some power under the alternative. Illustration of the potential problem: generating a p-value as a uniform distribution on 0 to 1 and rejecting when $p leq alpha$ is a (admittedly pretty useless) level $alpha$ test for any null hypothesis, but you will get a uniform distribution of p-values whether $H_0$ is true or not.
- You can only really show that you are quite close to $H_0$ being true (i.e. under the true parameter values three distribution might be close to uniform, even if $H_0$ is false.
With realistic applications, you tend to get additional issues. These mostly arise, because no one person/lab/study group can usually do all the necessary studies. As a result one tends to look at studies from lots of groups, at which point you have increased concerns (i.e. if you had done all relevant experiments yourself, at least you'd know) of underreporting, selective reporting of significant/surprising findings, p-hacking, multiple testing/multiple testing corrections and so on.
edited Nov 13 at 20:54
answered Nov 13 at 16:08


Björn
8,6411834
8,6411834
(+1) The power point is hugely important! Different theories can produce observationally equivalent data, and a critical part of experiment design is to produce and/or collect data that would allow you to distinguish.
– Matthew Gunn
Nov 15 at 16:35
add a comment |
(+1) The power point is hugely important! Different theories can produce observationally equivalent data, and a critical part of experiment design is to produce and/or collect data that would allow you to distinguish.
– Matthew Gunn
Nov 15 at 16:35
(+1) The power point is hugely important! Different theories can produce observationally equivalent data, and a critical part of experiment design is to produce and/or collect data that would allow you to distinguish.
– Matthew Gunn
Nov 15 at 16:35
(+1) The power point is hugely important! Different theories can produce observationally equivalent data, and a critical part of experiment design is to produce and/or collect data that would allow you to distinguish.
– Matthew Gunn
Nov 15 at 16:35
add a comment |
up vote
-2
down vote
Null hypothesis (H0): Gravity causes everything in the universe to fall toward Earth's surface.
Alternate hypothesis (H1): Nothing ever falls.
Performed 1 million experiments with dozens of household objects, fail to reject H0 with $p < 0.01$ every time. Is H0 true?
New contributor
usul is a new contributor to this site. Take care in asking for clarification, commenting, and answering.
Check out our Code of Conduct.
2
Do you think Galileo did one million trials? None of this stuff is necessary in physical sciences. Establishing the laws of nature by applying scientific method does not reduce into statistical inference.
– Aksakal
Nov 13 at 20:11
1
-1 This is scientifically, statistically, and historically inaccurate. Greeks once believed that it was affinity that drew objects to the Earth. Not bad, but doesn't explain the 3+ body system problems well. Hypotheses should be complementary. Lastly stating a possibly known bias as H_0 and showing experiments continue to lead to the same incorrect conclusion doesn't make the conclusion correct. e.g. women earn less than men b/c they are less driven, sample all women's salaries, H_0 is true!
– AdamO
Nov 13 at 21:00
@AdamO that is exactly my point.
– usul
Nov 13 at 21:01
@AdamO, in the Western countries women earn less when they work less for a variety of reasons including their own choice, disincentives of all kinds and hostile work environment in some places. When they work the same, they earn about the same, e.g. see medicare nurse salaries where women are the great majority: medscape.com/slideshow/…. They all earn the same $37 when working hourly. Totally off-topic, of course.
– Aksakal
Nov 13 at 21:27
2
If your null hypothesis isGravity causes everything in the universe to fall toward Earth's surface
isn't the alternative hypothesisThere is at least one thing in the universe that does not fall toward the Earth's surface
and notNothing ever falls
?
– Eff
Nov 14 at 8:13
|
show 2 more comments
up vote
-2
down vote
Null hypothesis (H0): Gravity causes everything in the universe to fall toward Earth's surface.
Alternate hypothesis (H1): Nothing ever falls.
Performed 1 million experiments with dozens of household objects, fail to reject H0 with $p < 0.01$ every time. Is H0 true?
New contributor
usul is a new contributor to this site. Take care in asking for clarification, commenting, and answering.
Check out our Code of Conduct.
2
Do you think Galileo did one million trials? None of this stuff is necessary in physical sciences. Establishing the laws of nature by applying scientific method does not reduce into statistical inference.
– Aksakal
Nov 13 at 20:11
1
-1 This is scientifically, statistically, and historically inaccurate. Greeks once believed that it was affinity that drew objects to the Earth. Not bad, but doesn't explain the 3+ body system problems well. Hypotheses should be complementary. Lastly stating a possibly known bias as H_0 and showing experiments continue to lead to the same incorrect conclusion doesn't make the conclusion correct. e.g. women earn less than men b/c they are less driven, sample all women's salaries, H_0 is true!
– AdamO
Nov 13 at 21:00
@AdamO that is exactly my point.
– usul
Nov 13 at 21:01
@AdamO, in the Western countries women earn less when they work less for a variety of reasons including their own choice, disincentives of all kinds and hostile work environment in some places. When they work the same, they earn about the same, e.g. see medicare nurse salaries where women are the great majority: medscape.com/slideshow/…. They all earn the same $37 when working hourly. Totally off-topic, of course.
– Aksakal
Nov 13 at 21:27
2
If your null hypothesis isGravity causes everything in the universe to fall toward Earth's surface
isn't the alternative hypothesisThere is at least one thing in the universe that does not fall toward the Earth's surface
and notNothing ever falls
?
– Eff
Nov 14 at 8:13
|
show 2 more comments
up vote
-2
down vote
up vote
-2
down vote
Null hypothesis (H0): Gravity causes everything in the universe to fall toward Earth's surface.
Alternate hypothesis (H1): Nothing ever falls.
Performed 1 million experiments with dozens of household objects, fail to reject H0 with $p < 0.01$ every time. Is H0 true?
New contributor
usul is a new contributor to this site. Take care in asking for clarification, commenting, and answering.
Check out our Code of Conduct.
Null hypothesis (H0): Gravity causes everything in the universe to fall toward Earth's surface.
Alternate hypothesis (H1): Nothing ever falls.
Performed 1 million experiments with dozens of household objects, fail to reject H0 with $p < 0.01$ every time. Is H0 true?
New contributor
usul is a new contributor to this site. Take care in asking for clarification, commenting, and answering.
Check out our Code of Conduct.
New contributor
usul is a new contributor to this site. Take care in asking for clarification, commenting, and answering.
Check out our Code of Conduct.
answered Nov 13 at 20:04
usul
971
971
New contributor
usul is a new contributor to this site. Take care in asking for clarification, commenting, and answering.
Check out our Code of Conduct.
New contributor
usul is a new contributor to this site. Take care in asking for clarification, commenting, and answering.
Check out our Code of Conduct.
usul is a new contributor to this site. Take care in asking for clarification, commenting, and answering.
Check out our Code of Conduct.
2
Do you think Galileo did one million trials? None of this stuff is necessary in physical sciences. Establishing the laws of nature by applying scientific method does not reduce into statistical inference.
– Aksakal
Nov 13 at 20:11
1
-1 This is scientifically, statistically, and historically inaccurate. Greeks once believed that it was affinity that drew objects to the Earth. Not bad, but doesn't explain the 3+ body system problems well. Hypotheses should be complementary. Lastly stating a possibly known bias as H_0 and showing experiments continue to lead to the same incorrect conclusion doesn't make the conclusion correct. e.g. women earn less than men b/c they are less driven, sample all women's salaries, H_0 is true!
– AdamO
Nov 13 at 21:00
@AdamO that is exactly my point.
– usul
Nov 13 at 21:01
@AdamO, in the Western countries women earn less when they work less for a variety of reasons including their own choice, disincentives of all kinds and hostile work environment in some places. When they work the same, they earn about the same, e.g. see medicare nurse salaries where women are the great majority: medscape.com/slideshow/…. They all earn the same $37 when working hourly. Totally off-topic, of course.
– Aksakal
Nov 13 at 21:27
2
If your null hypothesis isGravity causes everything in the universe to fall toward Earth's surface
isn't the alternative hypothesisThere is at least one thing in the universe that does not fall toward the Earth's surface
and notNothing ever falls
?
– Eff
Nov 14 at 8:13
|
show 2 more comments
2
Do you think Galileo did one million trials? None of this stuff is necessary in physical sciences. Establishing the laws of nature by applying scientific method does not reduce into statistical inference.
– Aksakal
Nov 13 at 20:11
1
-1 This is scientifically, statistically, and historically inaccurate. Greeks once believed that it was affinity that drew objects to the Earth. Not bad, but doesn't explain the 3+ body system problems well. Hypotheses should be complementary. Lastly stating a possibly known bias as H_0 and showing experiments continue to lead to the same incorrect conclusion doesn't make the conclusion correct. e.g. women earn less than men b/c they are less driven, sample all women's salaries, H_0 is true!
– AdamO
Nov 13 at 21:00
@AdamO that is exactly my point.
– usul
Nov 13 at 21:01
@AdamO, in the Western countries women earn less when they work less for a variety of reasons including their own choice, disincentives of all kinds and hostile work environment in some places. When they work the same, they earn about the same, e.g. see medicare nurse salaries where women are the great majority: medscape.com/slideshow/…. They all earn the same $37 when working hourly. Totally off-topic, of course.
– Aksakal
Nov 13 at 21:27
2
If your null hypothesis isGravity causes everything in the universe to fall toward Earth's surface
isn't the alternative hypothesisThere is at least one thing in the universe that does not fall toward the Earth's surface
and notNothing ever falls
?
– Eff
Nov 14 at 8:13
2
2
Do you think Galileo did one million trials? None of this stuff is necessary in physical sciences. Establishing the laws of nature by applying scientific method does not reduce into statistical inference.
– Aksakal
Nov 13 at 20:11
Do you think Galileo did one million trials? None of this stuff is necessary in physical sciences. Establishing the laws of nature by applying scientific method does not reduce into statistical inference.
– Aksakal
Nov 13 at 20:11
1
1
-1 This is scientifically, statistically, and historically inaccurate. Greeks once believed that it was affinity that drew objects to the Earth. Not bad, but doesn't explain the 3+ body system problems well. Hypotheses should be complementary. Lastly stating a possibly known bias as H_0 and showing experiments continue to lead to the same incorrect conclusion doesn't make the conclusion correct. e.g. women earn less than men b/c they are less driven, sample all women's salaries, H_0 is true!
– AdamO
Nov 13 at 21:00
-1 This is scientifically, statistically, and historically inaccurate. Greeks once believed that it was affinity that drew objects to the Earth. Not bad, but doesn't explain the 3+ body system problems well. Hypotheses should be complementary. Lastly stating a possibly known bias as H_0 and showing experiments continue to lead to the same incorrect conclusion doesn't make the conclusion correct. e.g. women earn less than men b/c they are less driven, sample all women's salaries, H_0 is true!
– AdamO
Nov 13 at 21:00
@AdamO that is exactly my point.
– usul
Nov 13 at 21:01
@AdamO that is exactly my point.
– usul
Nov 13 at 21:01
@AdamO, in the Western countries women earn less when they work less for a variety of reasons including their own choice, disincentives of all kinds and hostile work environment in some places. When they work the same, they earn about the same, e.g. see medicare nurse salaries where women are the great majority: medscape.com/slideshow/…. They all earn the same $37 when working hourly. Totally off-topic, of course.
– Aksakal
Nov 13 at 21:27
@AdamO, in the Western countries women earn less when they work less for a variety of reasons including their own choice, disincentives of all kinds and hostile work environment in some places. When they work the same, they earn about the same, e.g. see medicare nurse salaries where women are the great majority: medscape.com/slideshow/…. They all earn the same $37 when working hourly. Totally off-topic, of course.
– Aksakal
Nov 13 at 21:27
2
2
If your null hypothesis is
Gravity causes everything in the universe to fall toward Earth's surface
isn't the alternative hypothesis There is at least one thing in the universe that does not fall toward the Earth's surface
and not Nothing ever falls
?– Eff
Nov 14 at 8:13
If your null hypothesis is
Gravity causes everything in the universe to fall toward Earth's surface
isn't the alternative hypothesis There is at least one thing in the universe that does not fall toward the Earth's surface
and not Nothing ever falls
?– Eff
Nov 14 at 8:13
|
show 2 more comments
Sign up or log in
StackExchange.ready(function () {
StackExchange.helpers.onClickDraftSave('#login-link');
});
Sign up using Google
Sign up using Facebook
Sign up using Email and Password
Post as a guest
Required, but never shown
StackExchange.ready(
function () {
StackExchange.openid.initPostLogin('.new-post-login', 'https%3a%2f%2fstats.stackexchange.com%2fquestions%2f376772%2fdoes-a-uniform-distribution-of-many-p-values-give-statistical-evidence-that-h0-i%23new-answer', 'question_page');
}
);
Post as a guest
Required, but never shown
Sign up or log in
StackExchange.ready(function () {
StackExchange.helpers.onClickDraftSave('#login-link');
});
Sign up using Google
Sign up using Facebook
Sign up using Email and Password
Post as a guest
Required, but never shown
Sign up or log in
StackExchange.ready(function () {
StackExchange.helpers.onClickDraftSave('#login-link');
});
Sign up using Google
Sign up using Facebook
Sign up using Email and Password
Post as a guest
Required, but never shown
Sign up or log in
StackExchange.ready(function () {
StackExchange.helpers.onClickDraftSave('#login-link');
});
Sign up using Google
Sign up using Facebook
Sign up using Email and Password
Sign up using Google
Sign up using Facebook
Sign up using Email and Password
Post as a guest
Required, but never shown
Required, but never shown
Required, but never shown
Required, but never shown
Required, but never shown
Required, but never shown
Required, but never shown
Required, but never shown
Required, but never shown
NxTjBRuyE3fGGC,ZpCUVXFHA4owMTwKIEgOqoFlwWHg,USzLRbhIXezP wyYeOtX aqsmCrh2NahvUKY,vQ8tWCv gE1 Jlv,KEt,7Y7L
1
You might be interested in my answer to a different question stats.stackexchange.com/questions/171742/… which has some comments about the hypotheses here.
– mdewey
Nov 13 at 15:59
H0 is false by its definition.
– Joshua
Nov 13 at 17:18
1
On a side note, the reason why i have so many tests (and haven't just combined all the data into a single one) is that my data is spatially distributed around the globe and i wanted to see whether there are spatial patterns in the p-values (there aren't, but if there were it would mean that either independence is violated or that H0/H1 is true in different parts of the globe). I haven't included this in the question text because i wanted to keep it general.
– Leander Moesinger
Nov 13 at 18:20