Understanding a specific step in the Feynman-Kac theorem proof
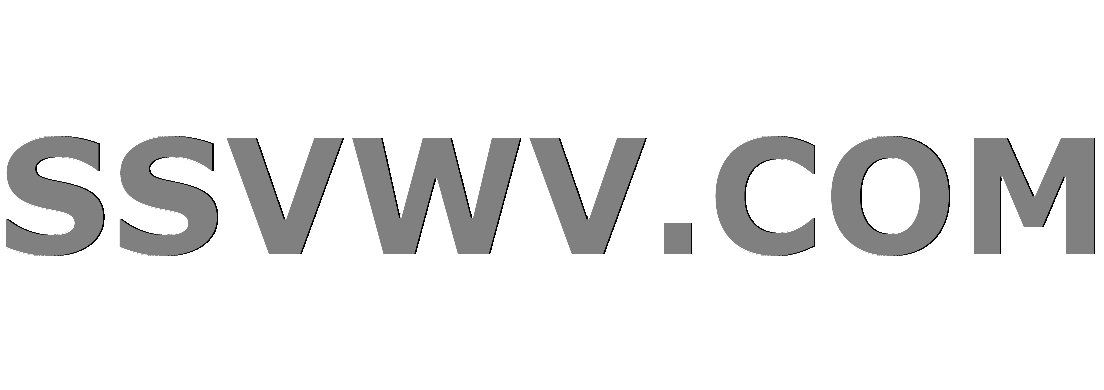
Multi tool use
up vote
2
down vote
favorite
I was looking at a proof of the Feynman-Kac theorem and I don't really understand a passage. The theorem is as follows
Let $k$ be a piecewise continuous function, $g in C^2(mathbb{R})$ and $B(t),t>0$ a standard Brownian motion. Then $$w(x,t) = Eleft{g(B(t))expleft{-int_0^t k(B(s))dsright}vert B(0)=x right}$$
is a unique solution to the following Cauchy problem $$left{begin{matrix}
frac{partial w}{partial t} = frac{1}{2}frac{partial^2 w}{partial t^2} -kw quad\
w(x,0)=g(x)
end{matrix}right.$$
for $xinmathbb{R}$ and $t>0$.
One of the proofs I've seen of this result is the following.
Consider the following identity
$$g(B(t))expleft{-int_0^t k(B(s))dsright} = \
g(B(t))expleft{-int_{Delta t}^t k(B(s))dsright} + \
g(B(t))expleft{-int_{Delta t}^t k(B(s))dsright}left(expleft{-int_0^{Delta t} k(B(s))dsright} -1right)$$
Now, keeping in mind the strong Markov property of Brownian motion and letting $mathcal{A}_{Delta t} = sigma{B(s), s leq Delta t }$, we have that
$$w(x,t) = \
Eleft{Eleft{g(B(t))expleft{-int_{Delta t}^t k(B(s))dsright} | mathcal{A}_{Delta t}right}right} + \
Eleft{Eleft{g(B(t))expleft{-int_{Delta t}^t k(B(s))dsright}left(expleft{-int_0^{Delta t} k(B(s))dsright} -1right) | mathcal{A}_{Delta t}right}right}$$
(in this step we just apply the law of conditional expectation)
Following on, the last expression equals
$(1)$ $$w(x,t) = \
Eleft{Eleft{ g(B(t-Delta t) e ^{-int_0^{t-Delta t}k(B(s))ds}right}| B(Delta t) + xright} + \
Eleft{ left( e^{-int_0^{Delta t}k(B(s))ds} -1right)Eleft{ g(B(t-Delta t))e^{-int_0^{t-Delta t}kB(s))ds}|B(Delta t) +x right}right}$$
which can be written as
$(2)$ $$E{ w(x+B(Delta t), t - Delta t)} + Eleft{left( e^{-int_0^{Delta t}k(B(s))ds} -1right)w(x+B(Delta t), t - Delta t) right} = \
Eleft{ left( w(x,t) + B(Delta t)frac{partial w}{partial x} + frac{1}{2}B^2(Delta t) frac{partial^2 w}{partial x^2}-Delta t frac{partial w}{partial t}right) (1-Delta tk(x))right} + o(Delta t)=\
w(x,t) + frac{1}{2}Delta tfrac{partial^2 w}{partial x^2} - Delta tfrac{partial w}{partial t} - Delta t k(x)w + o(Delta t)$$
dividing everything by $Delta t$ and letting $Delta t rightarrow 0$ we show that $w$ satisfies the starting Cauchy problem.
Excuse me for the clumpiness of the notation. Now, to the questions
$a)$ in $(1)$, how exactly was the strong Markov property of Brownian motion used? I know that it states that
$$ P(B(t) in A|mathcal{F}_s) = P(B(t) in A | B(s)) quad forall tgeq s quad A in mathcal{B}(mathbb{R})$$
so I can see how the conditioning to the $sigma$-algebra was dropped, but what justifies the time shift in the Brownian motion and in the integral bounds?
$b)$ in $(2)$, how is that series expansion made? I can see how it is done for the first summand, but how is it done for the second summand?
stochastic-processes proof-explanation brownian-motion
add a comment |
up vote
2
down vote
favorite
I was looking at a proof of the Feynman-Kac theorem and I don't really understand a passage. The theorem is as follows
Let $k$ be a piecewise continuous function, $g in C^2(mathbb{R})$ and $B(t),t>0$ a standard Brownian motion. Then $$w(x,t) = Eleft{g(B(t))expleft{-int_0^t k(B(s))dsright}vert B(0)=x right}$$
is a unique solution to the following Cauchy problem $$left{begin{matrix}
frac{partial w}{partial t} = frac{1}{2}frac{partial^2 w}{partial t^2} -kw quad\
w(x,0)=g(x)
end{matrix}right.$$
for $xinmathbb{R}$ and $t>0$.
One of the proofs I've seen of this result is the following.
Consider the following identity
$$g(B(t))expleft{-int_0^t k(B(s))dsright} = \
g(B(t))expleft{-int_{Delta t}^t k(B(s))dsright} + \
g(B(t))expleft{-int_{Delta t}^t k(B(s))dsright}left(expleft{-int_0^{Delta t} k(B(s))dsright} -1right)$$
Now, keeping in mind the strong Markov property of Brownian motion and letting $mathcal{A}_{Delta t} = sigma{B(s), s leq Delta t }$, we have that
$$w(x,t) = \
Eleft{Eleft{g(B(t))expleft{-int_{Delta t}^t k(B(s))dsright} | mathcal{A}_{Delta t}right}right} + \
Eleft{Eleft{g(B(t))expleft{-int_{Delta t}^t k(B(s))dsright}left(expleft{-int_0^{Delta t} k(B(s))dsright} -1right) | mathcal{A}_{Delta t}right}right}$$
(in this step we just apply the law of conditional expectation)
Following on, the last expression equals
$(1)$ $$w(x,t) = \
Eleft{Eleft{ g(B(t-Delta t) e ^{-int_0^{t-Delta t}k(B(s))ds}right}| B(Delta t) + xright} + \
Eleft{ left( e^{-int_0^{Delta t}k(B(s))ds} -1right)Eleft{ g(B(t-Delta t))e^{-int_0^{t-Delta t}kB(s))ds}|B(Delta t) +x right}right}$$
which can be written as
$(2)$ $$E{ w(x+B(Delta t), t - Delta t)} + Eleft{left( e^{-int_0^{Delta t}k(B(s))ds} -1right)w(x+B(Delta t), t - Delta t) right} = \
Eleft{ left( w(x,t) + B(Delta t)frac{partial w}{partial x} + frac{1}{2}B^2(Delta t) frac{partial^2 w}{partial x^2}-Delta t frac{partial w}{partial t}right) (1-Delta tk(x))right} + o(Delta t)=\
w(x,t) + frac{1}{2}Delta tfrac{partial^2 w}{partial x^2} - Delta tfrac{partial w}{partial t} - Delta t k(x)w + o(Delta t)$$
dividing everything by $Delta t$ and letting $Delta t rightarrow 0$ we show that $w$ satisfies the starting Cauchy problem.
Excuse me for the clumpiness of the notation. Now, to the questions
$a)$ in $(1)$, how exactly was the strong Markov property of Brownian motion used? I know that it states that
$$ P(B(t) in A|mathcal{F}_s) = P(B(t) in A | B(s)) quad forall tgeq s quad A in mathcal{B}(mathbb{R})$$
so I can see how the conditioning to the $sigma$-algebra was dropped, but what justifies the time shift in the Brownian motion and in the integral bounds?
$b)$ in $(2)$, how is that series expansion made? I can see how it is done for the first summand, but how is it done for the second summand?
stochastic-processes proof-explanation brownian-motion
add a comment |
up vote
2
down vote
favorite
up vote
2
down vote
favorite
I was looking at a proof of the Feynman-Kac theorem and I don't really understand a passage. The theorem is as follows
Let $k$ be a piecewise continuous function, $g in C^2(mathbb{R})$ and $B(t),t>0$ a standard Brownian motion. Then $$w(x,t) = Eleft{g(B(t))expleft{-int_0^t k(B(s))dsright}vert B(0)=x right}$$
is a unique solution to the following Cauchy problem $$left{begin{matrix}
frac{partial w}{partial t} = frac{1}{2}frac{partial^2 w}{partial t^2} -kw quad\
w(x,0)=g(x)
end{matrix}right.$$
for $xinmathbb{R}$ and $t>0$.
One of the proofs I've seen of this result is the following.
Consider the following identity
$$g(B(t))expleft{-int_0^t k(B(s))dsright} = \
g(B(t))expleft{-int_{Delta t}^t k(B(s))dsright} + \
g(B(t))expleft{-int_{Delta t}^t k(B(s))dsright}left(expleft{-int_0^{Delta t} k(B(s))dsright} -1right)$$
Now, keeping in mind the strong Markov property of Brownian motion and letting $mathcal{A}_{Delta t} = sigma{B(s), s leq Delta t }$, we have that
$$w(x,t) = \
Eleft{Eleft{g(B(t))expleft{-int_{Delta t}^t k(B(s))dsright} | mathcal{A}_{Delta t}right}right} + \
Eleft{Eleft{g(B(t))expleft{-int_{Delta t}^t k(B(s))dsright}left(expleft{-int_0^{Delta t} k(B(s))dsright} -1right) | mathcal{A}_{Delta t}right}right}$$
(in this step we just apply the law of conditional expectation)
Following on, the last expression equals
$(1)$ $$w(x,t) = \
Eleft{Eleft{ g(B(t-Delta t) e ^{-int_0^{t-Delta t}k(B(s))ds}right}| B(Delta t) + xright} + \
Eleft{ left( e^{-int_0^{Delta t}k(B(s))ds} -1right)Eleft{ g(B(t-Delta t))e^{-int_0^{t-Delta t}kB(s))ds}|B(Delta t) +x right}right}$$
which can be written as
$(2)$ $$E{ w(x+B(Delta t), t - Delta t)} + Eleft{left( e^{-int_0^{Delta t}k(B(s))ds} -1right)w(x+B(Delta t), t - Delta t) right} = \
Eleft{ left( w(x,t) + B(Delta t)frac{partial w}{partial x} + frac{1}{2}B^2(Delta t) frac{partial^2 w}{partial x^2}-Delta t frac{partial w}{partial t}right) (1-Delta tk(x))right} + o(Delta t)=\
w(x,t) + frac{1}{2}Delta tfrac{partial^2 w}{partial x^2} - Delta tfrac{partial w}{partial t} - Delta t k(x)w + o(Delta t)$$
dividing everything by $Delta t$ and letting $Delta t rightarrow 0$ we show that $w$ satisfies the starting Cauchy problem.
Excuse me for the clumpiness of the notation. Now, to the questions
$a)$ in $(1)$, how exactly was the strong Markov property of Brownian motion used? I know that it states that
$$ P(B(t) in A|mathcal{F}_s) = P(B(t) in A | B(s)) quad forall tgeq s quad A in mathcal{B}(mathbb{R})$$
so I can see how the conditioning to the $sigma$-algebra was dropped, but what justifies the time shift in the Brownian motion and in the integral bounds?
$b)$ in $(2)$, how is that series expansion made? I can see how it is done for the first summand, but how is it done for the second summand?
stochastic-processes proof-explanation brownian-motion
I was looking at a proof of the Feynman-Kac theorem and I don't really understand a passage. The theorem is as follows
Let $k$ be a piecewise continuous function, $g in C^2(mathbb{R})$ and $B(t),t>0$ a standard Brownian motion. Then $$w(x,t) = Eleft{g(B(t))expleft{-int_0^t k(B(s))dsright}vert B(0)=x right}$$
is a unique solution to the following Cauchy problem $$left{begin{matrix}
frac{partial w}{partial t} = frac{1}{2}frac{partial^2 w}{partial t^2} -kw quad\
w(x,0)=g(x)
end{matrix}right.$$
for $xinmathbb{R}$ and $t>0$.
One of the proofs I've seen of this result is the following.
Consider the following identity
$$g(B(t))expleft{-int_0^t k(B(s))dsright} = \
g(B(t))expleft{-int_{Delta t}^t k(B(s))dsright} + \
g(B(t))expleft{-int_{Delta t}^t k(B(s))dsright}left(expleft{-int_0^{Delta t} k(B(s))dsright} -1right)$$
Now, keeping in mind the strong Markov property of Brownian motion and letting $mathcal{A}_{Delta t} = sigma{B(s), s leq Delta t }$, we have that
$$w(x,t) = \
Eleft{Eleft{g(B(t))expleft{-int_{Delta t}^t k(B(s))dsright} | mathcal{A}_{Delta t}right}right} + \
Eleft{Eleft{g(B(t))expleft{-int_{Delta t}^t k(B(s))dsright}left(expleft{-int_0^{Delta t} k(B(s))dsright} -1right) | mathcal{A}_{Delta t}right}right}$$
(in this step we just apply the law of conditional expectation)
Following on, the last expression equals
$(1)$ $$w(x,t) = \
Eleft{Eleft{ g(B(t-Delta t) e ^{-int_0^{t-Delta t}k(B(s))ds}right}| B(Delta t) + xright} + \
Eleft{ left( e^{-int_0^{Delta t}k(B(s))ds} -1right)Eleft{ g(B(t-Delta t))e^{-int_0^{t-Delta t}kB(s))ds}|B(Delta t) +x right}right}$$
which can be written as
$(2)$ $$E{ w(x+B(Delta t), t - Delta t)} + Eleft{left( e^{-int_0^{Delta t}k(B(s))ds} -1right)w(x+B(Delta t), t - Delta t) right} = \
Eleft{ left( w(x,t) + B(Delta t)frac{partial w}{partial x} + frac{1}{2}B^2(Delta t) frac{partial^2 w}{partial x^2}-Delta t frac{partial w}{partial t}right) (1-Delta tk(x))right} + o(Delta t)=\
w(x,t) + frac{1}{2}Delta tfrac{partial^2 w}{partial x^2} - Delta tfrac{partial w}{partial t} - Delta t k(x)w + o(Delta t)$$
dividing everything by $Delta t$ and letting $Delta t rightarrow 0$ we show that $w$ satisfies the starting Cauchy problem.
Excuse me for the clumpiness of the notation. Now, to the questions
$a)$ in $(1)$, how exactly was the strong Markov property of Brownian motion used? I know that it states that
$$ P(B(t) in A|mathcal{F}_s) = P(B(t) in A | B(s)) quad forall tgeq s quad A in mathcal{B}(mathbb{R})$$
so I can see how the conditioning to the $sigma$-algebra was dropped, but what justifies the time shift in the Brownian motion and in the integral bounds?
$b)$ in $(2)$, how is that series expansion made? I can see how it is done for the first summand, but how is it done for the second summand?
stochastic-processes proof-explanation brownian-motion
stochastic-processes proof-explanation brownian-motion
asked Nov 21 at 12:50
Easymode44
313111
313111
add a comment |
add a comment |
active
oldest
votes
active
oldest
votes
active
oldest
votes
active
oldest
votes
active
oldest
votes
Thanks for contributing an answer to Mathematics Stack Exchange!
- Please be sure to answer the question. Provide details and share your research!
But avoid …
- Asking for help, clarification, or responding to other answers.
- Making statements based on opinion; back them up with references or personal experience.
Use MathJax to format equations. MathJax reference.
To learn more, see our tips on writing great answers.
Some of your past answers have not been well-received, and you're in danger of being blocked from answering.
Please pay close attention to the following guidance:
- Please be sure to answer the question. Provide details and share your research!
But avoid …
- Asking for help, clarification, or responding to other answers.
- Making statements based on opinion; back them up with references or personal experience.
To learn more, see our tips on writing great answers.
Sign up or log in
StackExchange.ready(function () {
StackExchange.helpers.onClickDraftSave('#login-link');
});
Sign up using Google
Sign up using Facebook
Sign up using Email and Password
Post as a guest
Required, but never shown
StackExchange.ready(
function () {
StackExchange.openid.initPostLogin('.new-post-login', 'https%3a%2f%2fmath.stackexchange.com%2fquestions%2f3007690%2funderstanding-a-specific-step-in-the-feynman-kac-theorem-proof%23new-answer', 'question_page');
}
);
Post as a guest
Required, but never shown
Sign up or log in
StackExchange.ready(function () {
StackExchange.helpers.onClickDraftSave('#login-link');
});
Sign up using Google
Sign up using Facebook
Sign up using Email and Password
Post as a guest
Required, but never shown
Sign up or log in
StackExchange.ready(function () {
StackExchange.helpers.onClickDraftSave('#login-link');
});
Sign up using Google
Sign up using Facebook
Sign up using Email and Password
Post as a guest
Required, but never shown
Sign up or log in
StackExchange.ready(function () {
StackExchange.helpers.onClickDraftSave('#login-link');
});
Sign up using Google
Sign up using Facebook
Sign up using Email and Password
Sign up using Google
Sign up using Facebook
Sign up using Email and Password
Post as a guest
Required, but never shown
Required, but never shown
Required, but never shown
Required, but never shown
Required, but never shown
Required, but never shown
Required, but never shown
Required, but never shown
Required, but never shown
KyTy,EWVd aJi3RBwYj1CVki2cIO25qFd0RS8xNB