What is the reason that Student-t Distribution is used when the number of samples is small
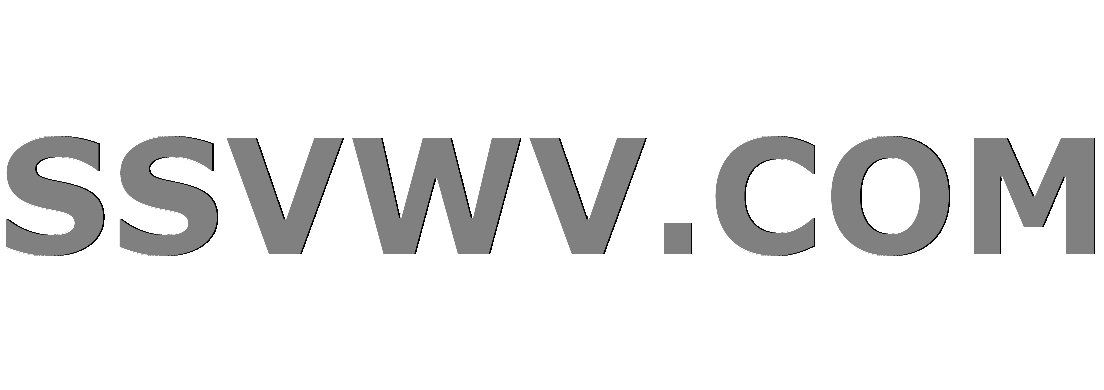
Multi tool use
$begingroup$
Let $bar{X}$ be the distribution of sample mean for $n$ identical and independent distributed as Normal distributions $N(mu, sigma^2)$.
The random variable
$$ frac{bar{X} - mu}{frac{sigma}{sqrt{n}}} $$
has standard normal distribution. Now let
$$ S^2 =frac{1}{n-1} sum_{i=1}^{n} (X_{i} - bar{X})^2, $$
then random variable
$$ frac{bar{X} - mu}{frac{S}{sqrt{n}}} $$
has student-t distribution with $n-1$ degrees of freedom.
From this, we can conclude then when $n$ is large, the random variable above will converge to the standard normal distribution,
$$ lim_{n rightarrow infty} S^2 = lim_{n rightarrow infty} frac{1}{n-1} sum_{i=1}^{n} (X_{i} - bar{X})^2 = lim_{n rightarrow infty} frac{1}{n} sum_{i=1}^{n} (X_{i} - mu)^2 = sigma^{2} $$
But why we should choose student-t distribution when the sample size is small..? What is the mathematical explanation..? thanks.
normal-distribution statistical-inference
$endgroup$
add a comment |
$begingroup$
Let $bar{X}$ be the distribution of sample mean for $n$ identical and independent distributed as Normal distributions $N(mu, sigma^2)$.
The random variable
$$ frac{bar{X} - mu}{frac{sigma}{sqrt{n}}} $$
has standard normal distribution. Now let
$$ S^2 =frac{1}{n-1} sum_{i=1}^{n} (X_{i} - bar{X})^2, $$
then random variable
$$ frac{bar{X} - mu}{frac{S}{sqrt{n}}} $$
has student-t distribution with $n-1$ degrees of freedom.
From this, we can conclude then when $n$ is large, the random variable above will converge to the standard normal distribution,
$$ lim_{n rightarrow infty} S^2 = lim_{n rightarrow infty} frac{1}{n-1} sum_{i=1}^{n} (X_{i} - bar{X})^2 = lim_{n rightarrow infty} frac{1}{n} sum_{i=1}^{n} (X_{i} - mu)^2 = sigma^{2} $$
But why we should choose student-t distribution when the sample size is small..? What is the mathematical explanation..? thanks.
normal-distribution statistical-inference
$endgroup$
$begingroup$
You provided the mathematical explanation already, namely $(bar{X}-mu)/frac{S}{sqrt{n}}sim t_{n-1}$.
$endgroup$
– user10354138
Nov 10 '18 at 7:51
$begingroup$
@user10354138 Yes, but that does not explain why we use student-t when $n$ is small..
$endgroup$
– Arief Anbiya
Nov 10 '18 at 7:55
$begingroup$
Why not? The question should be why we could avoid it when $n$ is large, not why we use it when $n$ is small.
$endgroup$
– user10354138
Nov 10 '18 at 7:57
$begingroup$
@user10354138 Is it because we have no other choices? and student-t resembles Normal distribution which makes it an approximation to the Normal distribution..?
$endgroup$
– Arief Anbiya
Nov 10 '18 at 7:59
add a comment |
$begingroup$
Let $bar{X}$ be the distribution of sample mean for $n$ identical and independent distributed as Normal distributions $N(mu, sigma^2)$.
The random variable
$$ frac{bar{X} - mu}{frac{sigma}{sqrt{n}}} $$
has standard normal distribution. Now let
$$ S^2 =frac{1}{n-1} sum_{i=1}^{n} (X_{i} - bar{X})^2, $$
then random variable
$$ frac{bar{X} - mu}{frac{S}{sqrt{n}}} $$
has student-t distribution with $n-1$ degrees of freedom.
From this, we can conclude then when $n$ is large, the random variable above will converge to the standard normal distribution,
$$ lim_{n rightarrow infty} S^2 = lim_{n rightarrow infty} frac{1}{n-1} sum_{i=1}^{n} (X_{i} - bar{X})^2 = lim_{n rightarrow infty} frac{1}{n} sum_{i=1}^{n} (X_{i} - mu)^2 = sigma^{2} $$
But why we should choose student-t distribution when the sample size is small..? What is the mathematical explanation..? thanks.
normal-distribution statistical-inference
$endgroup$
Let $bar{X}$ be the distribution of sample mean for $n$ identical and independent distributed as Normal distributions $N(mu, sigma^2)$.
The random variable
$$ frac{bar{X} - mu}{frac{sigma}{sqrt{n}}} $$
has standard normal distribution. Now let
$$ S^2 =frac{1}{n-1} sum_{i=1}^{n} (X_{i} - bar{X})^2, $$
then random variable
$$ frac{bar{X} - mu}{frac{S}{sqrt{n}}} $$
has student-t distribution with $n-1$ degrees of freedom.
From this, we can conclude then when $n$ is large, the random variable above will converge to the standard normal distribution,
$$ lim_{n rightarrow infty} S^2 = lim_{n rightarrow infty} frac{1}{n-1} sum_{i=1}^{n} (X_{i} - bar{X})^2 = lim_{n rightarrow infty} frac{1}{n} sum_{i=1}^{n} (X_{i} - mu)^2 = sigma^{2} $$
But why we should choose student-t distribution when the sample size is small..? What is the mathematical explanation..? thanks.
normal-distribution statistical-inference
normal-distribution statistical-inference
asked Nov 10 '18 at 7:25
Arief AnbiyaArief Anbiya
1,3601622
1,3601622
$begingroup$
You provided the mathematical explanation already, namely $(bar{X}-mu)/frac{S}{sqrt{n}}sim t_{n-1}$.
$endgroup$
– user10354138
Nov 10 '18 at 7:51
$begingroup$
@user10354138 Yes, but that does not explain why we use student-t when $n$ is small..
$endgroup$
– Arief Anbiya
Nov 10 '18 at 7:55
$begingroup$
Why not? The question should be why we could avoid it when $n$ is large, not why we use it when $n$ is small.
$endgroup$
– user10354138
Nov 10 '18 at 7:57
$begingroup$
@user10354138 Is it because we have no other choices? and student-t resembles Normal distribution which makes it an approximation to the Normal distribution..?
$endgroup$
– Arief Anbiya
Nov 10 '18 at 7:59
add a comment |
$begingroup$
You provided the mathematical explanation already, namely $(bar{X}-mu)/frac{S}{sqrt{n}}sim t_{n-1}$.
$endgroup$
– user10354138
Nov 10 '18 at 7:51
$begingroup$
@user10354138 Yes, but that does not explain why we use student-t when $n$ is small..
$endgroup$
– Arief Anbiya
Nov 10 '18 at 7:55
$begingroup$
Why not? The question should be why we could avoid it when $n$ is large, not why we use it when $n$ is small.
$endgroup$
– user10354138
Nov 10 '18 at 7:57
$begingroup$
@user10354138 Is it because we have no other choices? and student-t resembles Normal distribution which makes it an approximation to the Normal distribution..?
$endgroup$
– Arief Anbiya
Nov 10 '18 at 7:59
$begingroup$
You provided the mathematical explanation already, namely $(bar{X}-mu)/frac{S}{sqrt{n}}sim t_{n-1}$.
$endgroup$
– user10354138
Nov 10 '18 at 7:51
$begingroup$
You provided the mathematical explanation already, namely $(bar{X}-mu)/frac{S}{sqrt{n}}sim t_{n-1}$.
$endgroup$
– user10354138
Nov 10 '18 at 7:51
$begingroup$
@user10354138 Yes, but that does not explain why we use student-t when $n$ is small..
$endgroup$
– Arief Anbiya
Nov 10 '18 at 7:55
$begingroup$
@user10354138 Yes, but that does not explain why we use student-t when $n$ is small..
$endgroup$
– Arief Anbiya
Nov 10 '18 at 7:55
$begingroup$
Why not? The question should be why we could avoid it when $n$ is large, not why we use it when $n$ is small.
$endgroup$
– user10354138
Nov 10 '18 at 7:57
$begingroup$
Why not? The question should be why we could avoid it when $n$ is large, not why we use it when $n$ is small.
$endgroup$
– user10354138
Nov 10 '18 at 7:57
$begingroup$
@user10354138 Is it because we have no other choices? and student-t resembles Normal distribution which makes it an approximation to the Normal distribution..?
$endgroup$
– Arief Anbiya
Nov 10 '18 at 7:59
$begingroup$
@user10354138 Is it because we have no other choices? and student-t resembles Normal distribution which makes it an approximation to the Normal distribution..?
$endgroup$
– Arief Anbiya
Nov 10 '18 at 7:59
add a comment |
3 Answers
3
active
oldest
votes
$begingroup$
The t-test is based on a Student's t-distribution which is sensitive to the number of observations. Furthermore, a t-statistic is calculated for small sample sizes where you do not know the population standard deviation. Even in the case of a large sample, we likely do not "know" the population standard deviation, but there are some nice results that help here.
The law of large numbers (LLN) gives us the result that sample averages converge in probability to the population average (formally, $bar{x} to mu$ as $n to infty$). That is, that as the sample size increases, the sample average gets closer and closer to the population average.
The central limit theorem (CLT) describes how the distribution of the difference in the sample and population averages ($bar{x}-mu$) changes with respect to the sample size $n$. Ultimately, what it tells us is that for sufficiently large $n$, this distribution approximates the normal distribution, $N(0,sigma^2 / n)$. Manipulating this expression, we can show the following
$$ bar{x}-mu sim N(0,sigma^2 / n) implies Z = frac{bar{x}-mu}{sigma/sqrt{n}} sim N(0,1) $$
So, in summary, the reason we can use a normal distribution for large samples is due to these results regarding convergence in distribution. This convergence happens fairly quickly (typically $nge30$ is sufficient), but for smaller samples these results do not hold and the Student's t-distribution is more appropriate.
$endgroup$
add a comment |
$begingroup$
The $t$ distribution is exact for all $n$. Therefore, we must use it or an adequate approximation. The Normal distribution is only a valid approximation for large $n$. The exact pdf is $frac{Gamma (frac{nu+1}{2})}{sqrt{nupi}Gamma(frac{nu}{2})}(1+frac{x^2}{nu})^{-frac{nu+1}{2}}$ with $nu=n-1$. For large $n$, this approximates $sqrt{frac{Gamma (frac{nu}{2}+1)}{Gamma (frac{nu}{2})nupi}}exp-frac{x^2}{2}=frac{1}{sqrt{2pi}}exp-frac{x^2}{2}$, the $N(0,,1)$ pdf.
$endgroup$
add a comment |
$begingroup$
I would like to post my view relating to @Chris answer also.
By the Central Limit Theorem, for large $m$, random var $X_{i}$ with mean $mu$ and st.dev $sigma$ from any distribution ($X_{i}$s have same dist) will have $$ sqrt{m} frac{ bar{X} - mu }{ sigma } $$ converge to standard normal. Also, the random.var $bar{X} - mu$ will be normal with mean $0$.
Now.., if $X_{i}$s are normal, then distribution
$$ T = sqrt{m} frac{bar{X} - mu}{S} $$ has student-t distribution with $m-1$ degrees of freedom. But we can only approximate the CLT using student-t when $X_{i}$s are normal.
Now I have an argument:
If $X_{i}$s are from the same distribution (any dist) with mean $mu$, then $bar{X} - mu$ will converge to normal with mean $0$ as $m$ gets large. So that
$$ sqrt{m}frac{frac{sum (bar{X}_{i} - mu)}{m} - 0}{S} $$
will have student-t distribution, and we can rewrite it as
$$ sqrt{m}frac{frac{sum (bar{X}_{i} )}{m} - mu}{S} $$
and for large $m$, $frac{sum (bar{X}_{i} )}{m}$ will converger to $bar{X}$. So we can still use $T$ as approximation even when $X_{i}$s are not normal.
$endgroup$
add a comment |
Your Answer
StackExchange.ifUsing("editor", function () {
return StackExchange.using("mathjaxEditing", function () {
StackExchange.MarkdownEditor.creationCallbacks.add(function (editor, postfix) {
StackExchange.mathjaxEditing.prepareWmdForMathJax(editor, postfix, [["$", "$"], ["\\(","\\)"]]);
});
});
}, "mathjax-editing");
StackExchange.ready(function() {
var channelOptions = {
tags: "".split(" "),
id: "69"
};
initTagRenderer("".split(" "), "".split(" "), channelOptions);
StackExchange.using("externalEditor", function() {
// Have to fire editor after snippets, if snippets enabled
if (StackExchange.settings.snippets.snippetsEnabled) {
StackExchange.using("snippets", function() {
createEditor();
});
}
else {
createEditor();
}
});
function createEditor() {
StackExchange.prepareEditor({
heartbeatType: 'answer',
autoActivateHeartbeat: false,
convertImagesToLinks: true,
noModals: true,
showLowRepImageUploadWarning: true,
reputationToPostImages: 10,
bindNavPrevention: true,
postfix: "",
imageUploader: {
brandingHtml: "Powered by u003ca class="icon-imgur-white" href="https://imgur.com/"u003eu003c/au003e",
contentPolicyHtml: "User contributions licensed under u003ca href="https://creativecommons.org/licenses/by-sa/3.0/"u003ecc by-sa 3.0 with attribution requiredu003c/au003e u003ca href="https://stackoverflow.com/legal/content-policy"u003e(content policy)u003c/au003e",
allowUrls: true
},
noCode: true, onDemand: true,
discardSelector: ".discard-answer"
,immediatelyShowMarkdownHelp:true
});
}
});
Sign up or log in
StackExchange.ready(function () {
StackExchange.helpers.onClickDraftSave('#login-link');
});
Sign up using Google
Sign up using Facebook
Sign up using Email and Password
Post as a guest
Required, but never shown
StackExchange.ready(
function () {
StackExchange.openid.initPostLogin('.new-post-login', 'https%3a%2f%2fmath.stackexchange.com%2fquestions%2f2992333%2fwhat-is-the-reason-that-student-t-distribution-is-used-when-the-number-of-sample%23new-answer', 'question_page');
}
);
Post as a guest
Required, but never shown
3 Answers
3
active
oldest
votes
3 Answers
3
active
oldest
votes
active
oldest
votes
active
oldest
votes
$begingroup$
The t-test is based on a Student's t-distribution which is sensitive to the number of observations. Furthermore, a t-statistic is calculated for small sample sizes where you do not know the population standard deviation. Even in the case of a large sample, we likely do not "know" the population standard deviation, but there are some nice results that help here.
The law of large numbers (LLN) gives us the result that sample averages converge in probability to the population average (formally, $bar{x} to mu$ as $n to infty$). That is, that as the sample size increases, the sample average gets closer and closer to the population average.
The central limit theorem (CLT) describes how the distribution of the difference in the sample and population averages ($bar{x}-mu$) changes with respect to the sample size $n$. Ultimately, what it tells us is that for sufficiently large $n$, this distribution approximates the normal distribution, $N(0,sigma^2 / n)$. Manipulating this expression, we can show the following
$$ bar{x}-mu sim N(0,sigma^2 / n) implies Z = frac{bar{x}-mu}{sigma/sqrt{n}} sim N(0,1) $$
So, in summary, the reason we can use a normal distribution for large samples is due to these results regarding convergence in distribution. This convergence happens fairly quickly (typically $nge30$ is sufficient), but for smaller samples these results do not hold and the Student's t-distribution is more appropriate.
$endgroup$
add a comment |
$begingroup$
The t-test is based on a Student's t-distribution which is sensitive to the number of observations. Furthermore, a t-statistic is calculated for small sample sizes where you do not know the population standard deviation. Even in the case of a large sample, we likely do not "know" the population standard deviation, but there are some nice results that help here.
The law of large numbers (LLN) gives us the result that sample averages converge in probability to the population average (formally, $bar{x} to mu$ as $n to infty$). That is, that as the sample size increases, the sample average gets closer and closer to the population average.
The central limit theorem (CLT) describes how the distribution of the difference in the sample and population averages ($bar{x}-mu$) changes with respect to the sample size $n$. Ultimately, what it tells us is that for sufficiently large $n$, this distribution approximates the normal distribution, $N(0,sigma^2 / n)$. Manipulating this expression, we can show the following
$$ bar{x}-mu sim N(0,sigma^2 / n) implies Z = frac{bar{x}-mu}{sigma/sqrt{n}} sim N(0,1) $$
So, in summary, the reason we can use a normal distribution for large samples is due to these results regarding convergence in distribution. This convergence happens fairly quickly (typically $nge30$ is sufficient), but for smaller samples these results do not hold and the Student's t-distribution is more appropriate.
$endgroup$
add a comment |
$begingroup$
The t-test is based on a Student's t-distribution which is sensitive to the number of observations. Furthermore, a t-statistic is calculated for small sample sizes where you do not know the population standard deviation. Even in the case of a large sample, we likely do not "know" the population standard deviation, but there are some nice results that help here.
The law of large numbers (LLN) gives us the result that sample averages converge in probability to the population average (formally, $bar{x} to mu$ as $n to infty$). That is, that as the sample size increases, the sample average gets closer and closer to the population average.
The central limit theorem (CLT) describes how the distribution of the difference in the sample and population averages ($bar{x}-mu$) changes with respect to the sample size $n$. Ultimately, what it tells us is that for sufficiently large $n$, this distribution approximates the normal distribution, $N(0,sigma^2 / n)$. Manipulating this expression, we can show the following
$$ bar{x}-mu sim N(0,sigma^2 / n) implies Z = frac{bar{x}-mu}{sigma/sqrt{n}} sim N(0,1) $$
So, in summary, the reason we can use a normal distribution for large samples is due to these results regarding convergence in distribution. This convergence happens fairly quickly (typically $nge30$ is sufficient), but for smaller samples these results do not hold and the Student's t-distribution is more appropriate.
$endgroup$
The t-test is based on a Student's t-distribution which is sensitive to the number of observations. Furthermore, a t-statistic is calculated for small sample sizes where you do not know the population standard deviation. Even in the case of a large sample, we likely do not "know" the population standard deviation, but there are some nice results that help here.
The law of large numbers (LLN) gives us the result that sample averages converge in probability to the population average (formally, $bar{x} to mu$ as $n to infty$). That is, that as the sample size increases, the sample average gets closer and closer to the population average.
The central limit theorem (CLT) describes how the distribution of the difference in the sample and population averages ($bar{x}-mu$) changes with respect to the sample size $n$. Ultimately, what it tells us is that for sufficiently large $n$, this distribution approximates the normal distribution, $N(0,sigma^2 / n)$. Manipulating this expression, we can show the following
$$ bar{x}-mu sim N(0,sigma^2 / n) implies Z = frac{bar{x}-mu}{sigma/sqrt{n}} sim N(0,1) $$
So, in summary, the reason we can use a normal distribution for large samples is due to these results regarding convergence in distribution. This convergence happens fairly quickly (typically $nge30$ is sufficient), but for smaller samples these results do not hold and the Student's t-distribution is more appropriate.
answered Nov 10 '18 at 8:49
ChrisChris
362
362
add a comment |
add a comment |
$begingroup$
The $t$ distribution is exact for all $n$. Therefore, we must use it or an adequate approximation. The Normal distribution is only a valid approximation for large $n$. The exact pdf is $frac{Gamma (frac{nu+1}{2})}{sqrt{nupi}Gamma(frac{nu}{2})}(1+frac{x^2}{nu})^{-frac{nu+1}{2}}$ with $nu=n-1$. For large $n$, this approximates $sqrt{frac{Gamma (frac{nu}{2}+1)}{Gamma (frac{nu}{2})nupi}}exp-frac{x^2}{2}=frac{1}{sqrt{2pi}}exp-frac{x^2}{2}$, the $N(0,,1)$ pdf.
$endgroup$
add a comment |
$begingroup$
The $t$ distribution is exact for all $n$. Therefore, we must use it or an adequate approximation. The Normal distribution is only a valid approximation for large $n$. The exact pdf is $frac{Gamma (frac{nu+1}{2})}{sqrt{nupi}Gamma(frac{nu}{2})}(1+frac{x^2}{nu})^{-frac{nu+1}{2}}$ with $nu=n-1$. For large $n$, this approximates $sqrt{frac{Gamma (frac{nu}{2}+1)}{Gamma (frac{nu}{2})nupi}}exp-frac{x^2}{2}=frac{1}{sqrt{2pi}}exp-frac{x^2}{2}$, the $N(0,,1)$ pdf.
$endgroup$
add a comment |
$begingroup$
The $t$ distribution is exact for all $n$. Therefore, we must use it or an adequate approximation. The Normal distribution is only a valid approximation for large $n$. The exact pdf is $frac{Gamma (frac{nu+1}{2})}{sqrt{nupi}Gamma(frac{nu}{2})}(1+frac{x^2}{nu})^{-frac{nu+1}{2}}$ with $nu=n-1$. For large $n$, this approximates $sqrt{frac{Gamma (frac{nu}{2}+1)}{Gamma (frac{nu}{2})nupi}}exp-frac{x^2}{2}=frac{1}{sqrt{2pi}}exp-frac{x^2}{2}$, the $N(0,,1)$ pdf.
$endgroup$
The $t$ distribution is exact for all $n$. Therefore, we must use it or an adequate approximation. The Normal distribution is only a valid approximation for large $n$. The exact pdf is $frac{Gamma (frac{nu+1}{2})}{sqrt{nupi}Gamma(frac{nu}{2})}(1+frac{x^2}{nu})^{-frac{nu+1}{2}}$ with $nu=n-1$. For large $n$, this approximates $sqrt{frac{Gamma (frac{nu}{2}+1)}{Gamma (frac{nu}{2})nupi}}exp-frac{x^2}{2}=frac{1}{sqrt{2pi}}exp-frac{x^2}{2}$, the $N(0,,1)$ pdf.
answered Nov 10 '18 at 9:21
J.G.J.G.
26.1k22539
26.1k22539
add a comment |
add a comment |
$begingroup$
I would like to post my view relating to @Chris answer also.
By the Central Limit Theorem, for large $m$, random var $X_{i}$ with mean $mu$ and st.dev $sigma$ from any distribution ($X_{i}$s have same dist) will have $$ sqrt{m} frac{ bar{X} - mu }{ sigma } $$ converge to standard normal. Also, the random.var $bar{X} - mu$ will be normal with mean $0$.
Now.., if $X_{i}$s are normal, then distribution
$$ T = sqrt{m} frac{bar{X} - mu}{S} $$ has student-t distribution with $m-1$ degrees of freedom. But we can only approximate the CLT using student-t when $X_{i}$s are normal.
Now I have an argument:
If $X_{i}$s are from the same distribution (any dist) with mean $mu$, then $bar{X} - mu$ will converge to normal with mean $0$ as $m$ gets large. So that
$$ sqrt{m}frac{frac{sum (bar{X}_{i} - mu)}{m} - 0}{S} $$
will have student-t distribution, and we can rewrite it as
$$ sqrt{m}frac{frac{sum (bar{X}_{i} )}{m} - mu}{S} $$
and for large $m$, $frac{sum (bar{X}_{i} )}{m}$ will converger to $bar{X}$. So we can still use $T$ as approximation even when $X_{i}$s are not normal.
$endgroup$
add a comment |
$begingroup$
I would like to post my view relating to @Chris answer also.
By the Central Limit Theorem, for large $m$, random var $X_{i}$ with mean $mu$ and st.dev $sigma$ from any distribution ($X_{i}$s have same dist) will have $$ sqrt{m} frac{ bar{X} - mu }{ sigma } $$ converge to standard normal. Also, the random.var $bar{X} - mu$ will be normal with mean $0$.
Now.., if $X_{i}$s are normal, then distribution
$$ T = sqrt{m} frac{bar{X} - mu}{S} $$ has student-t distribution with $m-1$ degrees of freedom. But we can only approximate the CLT using student-t when $X_{i}$s are normal.
Now I have an argument:
If $X_{i}$s are from the same distribution (any dist) with mean $mu$, then $bar{X} - mu$ will converge to normal with mean $0$ as $m$ gets large. So that
$$ sqrt{m}frac{frac{sum (bar{X}_{i} - mu)}{m} - 0}{S} $$
will have student-t distribution, and we can rewrite it as
$$ sqrt{m}frac{frac{sum (bar{X}_{i} )}{m} - mu}{S} $$
and for large $m$, $frac{sum (bar{X}_{i} )}{m}$ will converger to $bar{X}$. So we can still use $T$ as approximation even when $X_{i}$s are not normal.
$endgroup$
add a comment |
$begingroup$
I would like to post my view relating to @Chris answer also.
By the Central Limit Theorem, for large $m$, random var $X_{i}$ with mean $mu$ and st.dev $sigma$ from any distribution ($X_{i}$s have same dist) will have $$ sqrt{m} frac{ bar{X} - mu }{ sigma } $$ converge to standard normal. Also, the random.var $bar{X} - mu$ will be normal with mean $0$.
Now.., if $X_{i}$s are normal, then distribution
$$ T = sqrt{m} frac{bar{X} - mu}{S} $$ has student-t distribution with $m-1$ degrees of freedom. But we can only approximate the CLT using student-t when $X_{i}$s are normal.
Now I have an argument:
If $X_{i}$s are from the same distribution (any dist) with mean $mu$, then $bar{X} - mu$ will converge to normal with mean $0$ as $m$ gets large. So that
$$ sqrt{m}frac{frac{sum (bar{X}_{i} - mu)}{m} - 0}{S} $$
will have student-t distribution, and we can rewrite it as
$$ sqrt{m}frac{frac{sum (bar{X}_{i} )}{m} - mu}{S} $$
and for large $m$, $frac{sum (bar{X}_{i} )}{m}$ will converger to $bar{X}$. So we can still use $T$ as approximation even when $X_{i}$s are not normal.
$endgroup$
I would like to post my view relating to @Chris answer also.
By the Central Limit Theorem, for large $m$, random var $X_{i}$ with mean $mu$ and st.dev $sigma$ from any distribution ($X_{i}$s have same dist) will have $$ sqrt{m} frac{ bar{X} - mu }{ sigma } $$ converge to standard normal. Also, the random.var $bar{X} - mu$ will be normal with mean $0$.
Now.., if $X_{i}$s are normal, then distribution
$$ T = sqrt{m} frac{bar{X} - mu}{S} $$ has student-t distribution with $m-1$ degrees of freedom. But we can only approximate the CLT using student-t when $X_{i}$s are normal.
Now I have an argument:
If $X_{i}$s are from the same distribution (any dist) with mean $mu$, then $bar{X} - mu$ will converge to normal with mean $0$ as $m$ gets large. So that
$$ sqrt{m}frac{frac{sum (bar{X}_{i} - mu)}{m} - 0}{S} $$
will have student-t distribution, and we can rewrite it as
$$ sqrt{m}frac{frac{sum (bar{X}_{i} )}{m} - mu}{S} $$
and for large $m$, $frac{sum (bar{X}_{i} )}{m}$ will converger to $bar{X}$. So we can still use $T$ as approximation even when $X_{i}$s are not normal.
answered Dec 16 '18 at 4:23
Arief AnbiyaArief Anbiya
1,3601622
1,3601622
add a comment |
add a comment |
Thanks for contributing an answer to Mathematics Stack Exchange!
- Please be sure to answer the question. Provide details and share your research!
But avoid …
- Asking for help, clarification, or responding to other answers.
- Making statements based on opinion; back them up with references or personal experience.
Use MathJax to format equations. MathJax reference.
To learn more, see our tips on writing great answers.
Sign up or log in
StackExchange.ready(function () {
StackExchange.helpers.onClickDraftSave('#login-link');
});
Sign up using Google
Sign up using Facebook
Sign up using Email and Password
Post as a guest
Required, but never shown
StackExchange.ready(
function () {
StackExchange.openid.initPostLogin('.new-post-login', 'https%3a%2f%2fmath.stackexchange.com%2fquestions%2f2992333%2fwhat-is-the-reason-that-student-t-distribution-is-used-when-the-number-of-sample%23new-answer', 'question_page');
}
);
Post as a guest
Required, but never shown
Sign up or log in
StackExchange.ready(function () {
StackExchange.helpers.onClickDraftSave('#login-link');
});
Sign up using Google
Sign up using Facebook
Sign up using Email and Password
Post as a guest
Required, but never shown
Sign up or log in
StackExchange.ready(function () {
StackExchange.helpers.onClickDraftSave('#login-link');
});
Sign up using Google
Sign up using Facebook
Sign up using Email and Password
Post as a guest
Required, but never shown
Sign up or log in
StackExchange.ready(function () {
StackExchange.helpers.onClickDraftSave('#login-link');
});
Sign up using Google
Sign up using Facebook
Sign up using Email and Password
Sign up using Google
Sign up using Facebook
Sign up using Email and Password
Post as a guest
Required, but never shown
Required, but never shown
Required, but never shown
Required, but never shown
Required, but never shown
Required, but never shown
Required, but never shown
Required, but never shown
Required, but never shown
1YEAug zCM 9FaeYmZZ,268vcv57Mrm,q1hQ4 MlcgncV mWIH28vRVB0K5jd,1Ls,W 8KH,YMouqldAvropO4D,4WTuO dIso
$begingroup$
You provided the mathematical explanation already, namely $(bar{X}-mu)/frac{S}{sqrt{n}}sim t_{n-1}$.
$endgroup$
– user10354138
Nov 10 '18 at 7:51
$begingroup$
@user10354138 Yes, but that does not explain why we use student-t when $n$ is small..
$endgroup$
– Arief Anbiya
Nov 10 '18 at 7:55
$begingroup$
Why not? The question should be why we could avoid it when $n$ is large, not why we use it when $n$ is small.
$endgroup$
– user10354138
Nov 10 '18 at 7:57
$begingroup$
@user10354138 Is it because we have no other choices? and student-t resembles Normal distribution which makes it an approximation to the Normal distribution..?
$endgroup$
– Arief Anbiya
Nov 10 '18 at 7:59