Probability that a random walk does not leave a subgraph after $k$ steps
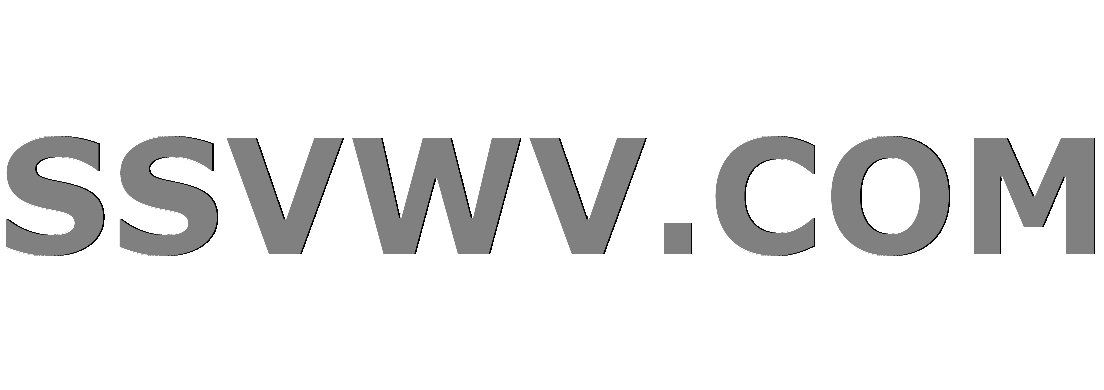
Multi tool use
$begingroup$
Suppose I have a connected, finite, graph $G = (V,E)$, and I have some vertex set $U$ such that the subgraph of $G$ induced by the vertices $Y = V setminus U$ is still connected.
Now, suppose I have a random walk $X_n$ on $G$, that follows the transition probabilities induced by the edge weights of $G$. Let $X_0 = u in Y$. I want to know how I can go about calculating $P_u(X_1, dots, X_k in Y)$.
I keep running into something like $prod_{i=1}^{k-1}P_u(X_{i+1} in W | X_{i}in W)$, which I don't know how to simplify either.
graph-theory markov-chains markov-process random-walk
$endgroup$
add a comment |
$begingroup$
Suppose I have a connected, finite, graph $G = (V,E)$, and I have some vertex set $U$ such that the subgraph of $G$ induced by the vertices $Y = V setminus U$ is still connected.
Now, suppose I have a random walk $X_n$ on $G$, that follows the transition probabilities induced by the edge weights of $G$. Let $X_0 = u in Y$. I want to know how I can go about calculating $P_u(X_1, dots, X_k in Y)$.
I keep running into something like $prod_{i=1}^{k-1}P_u(X_{i+1} in W | X_{i}in W)$, which I don't know how to simplify either.
graph-theory markov-chains markov-process random-walk
$endgroup$
add a comment |
$begingroup$
Suppose I have a connected, finite, graph $G = (V,E)$, and I have some vertex set $U$ such that the subgraph of $G$ induced by the vertices $Y = V setminus U$ is still connected.
Now, suppose I have a random walk $X_n$ on $G$, that follows the transition probabilities induced by the edge weights of $G$. Let $X_0 = u in Y$. I want to know how I can go about calculating $P_u(X_1, dots, X_k in Y)$.
I keep running into something like $prod_{i=1}^{k-1}P_u(X_{i+1} in W | X_{i}in W)$, which I don't know how to simplify either.
graph-theory markov-chains markov-process random-walk
$endgroup$
Suppose I have a connected, finite, graph $G = (V,E)$, and I have some vertex set $U$ such that the subgraph of $G$ induced by the vertices $Y = V setminus U$ is still connected.
Now, suppose I have a random walk $X_n$ on $G$, that follows the transition probabilities induced by the edge weights of $G$. Let $X_0 = u in Y$. I want to know how I can go about calculating $P_u(X_1, dots, X_k in Y)$.
I keep running into something like $prod_{i=1}^{k-1}P_u(X_{i+1} in W | X_{i}in W)$, which I don't know how to simplify either.
graph-theory markov-chains markov-process random-walk
graph-theory markov-chains markov-process random-walk
asked Dec 1 '18 at 18:05
jackson5jackson5
606512
606512
add a comment |
add a comment |
1 Answer
1
active
oldest
votes
$begingroup$
To simplify the problem as much as possible, you can combine all vertices in $U$ into a single vertex $x$. Each edge from $v in Y$ to a vertex in $U$ becomes an edge from $v$ to $x$; if you get parallel edges this way, you should either keep them both, or else combine them by adding the weights.
Next, turn the random walk into an asymmetric Markov chain by making $x$ an absorbing state. This will make it easy to tell when we've left $Y$: if we do, we go to $x$, and then we stay in $x$ forever.
Now, the complementary probability (the probability that you get from your starting vertex $u$ to $x$ within $k$ steps) can be computed by taking powers of the transition matrix of this Markov chain, as usual.
$endgroup$
add a comment |
Your Answer
StackExchange.ifUsing("editor", function () {
return StackExchange.using("mathjaxEditing", function () {
StackExchange.MarkdownEditor.creationCallbacks.add(function (editor, postfix) {
StackExchange.mathjaxEditing.prepareWmdForMathJax(editor, postfix, [["$", "$"], ["\\(","\\)"]]);
});
});
}, "mathjax-editing");
StackExchange.ready(function() {
var channelOptions = {
tags: "".split(" "),
id: "69"
};
initTagRenderer("".split(" "), "".split(" "), channelOptions);
StackExchange.using("externalEditor", function() {
// Have to fire editor after snippets, if snippets enabled
if (StackExchange.settings.snippets.snippetsEnabled) {
StackExchange.using("snippets", function() {
createEditor();
});
}
else {
createEditor();
}
});
function createEditor() {
StackExchange.prepareEditor({
heartbeatType: 'answer',
autoActivateHeartbeat: false,
convertImagesToLinks: true,
noModals: true,
showLowRepImageUploadWarning: true,
reputationToPostImages: 10,
bindNavPrevention: true,
postfix: "",
imageUploader: {
brandingHtml: "Powered by u003ca class="icon-imgur-white" href="https://imgur.com/"u003eu003c/au003e",
contentPolicyHtml: "User contributions licensed under u003ca href="https://creativecommons.org/licenses/by-sa/3.0/"u003ecc by-sa 3.0 with attribution requiredu003c/au003e u003ca href="https://stackoverflow.com/legal/content-policy"u003e(content policy)u003c/au003e",
allowUrls: true
},
noCode: true, onDemand: true,
discardSelector: ".discard-answer"
,immediatelyShowMarkdownHelp:true
});
}
});
Sign up or log in
StackExchange.ready(function () {
StackExchange.helpers.onClickDraftSave('#login-link');
});
Sign up using Google
Sign up using Facebook
Sign up using Email and Password
Post as a guest
Required, but never shown
StackExchange.ready(
function () {
StackExchange.openid.initPostLogin('.new-post-login', 'https%3a%2f%2fmath.stackexchange.com%2fquestions%2f3021633%2fprobability-that-a-random-walk-does-not-leave-a-subgraph-after-k-steps%23new-answer', 'question_page');
}
);
Post as a guest
Required, but never shown
1 Answer
1
active
oldest
votes
1 Answer
1
active
oldest
votes
active
oldest
votes
active
oldest
votes
$begingroup$
To simplify the problem as much as possible, you can combine all vertices in $U$ into a single vertex $x$. Each edge from $v in Y$ to a vertex in $U$ becomes an edge from $v$ to $x$; if you get parallel edges this way, you should either keep them both, or else combine them by adding the weights.
Next, turn the random walk into an asymmetric Markov chain by making $x$ an absorbing state. This will make it easy to tell when we've left $Y$: if we do, we go to $x$, and then we stay in $x$ forever.
Now, the complementary probability (the probability that you get from your starting vertex $u$ to $x$ within $k$ steps) can be computed by taking powers of the transition matrix of this Markov chain, as usual.
$endgroup$
add a comment |
$begingroup$
To simplify the problem as much as possible, you can combine all vertices in $U$ into a single vertex $x$. Each edge from $v in Y$ to a vertex in $U$ becomes an edge from $v$ to $x$; if you get parallel edges this way, you should either keep them both, or else combine them by adding the weights.
Next, turn the random walk into an asymmetric Markov chain by making $x$ an absorbing state. This will make it easy to tell when we've left $Y$: if we do, we go to $x$, and then we stay in $x$ forever.
Now, the complementary probability (the probability that you get from your starting vertex $u$ to $x$ within $k$ steps) can be computed by taking powers of the transition matrix of this Markov chain, as usual.
$endgroup$
add a comment |
$begingroup$
To simplify the problem as much as possible, you can combine all vertices in $U$ into a single vertex $x$. Each edge from $v in Y$ to a vertex in $U$ becomes an edge from $v$ to $x$; if you get parallel edges this way, you should either keep them both, or else combine them by adding the weights.
Next, turn the random walk into an asymmetric Markov chain by making $x$ an absorbing state. This will make it easy to tell when we've left $Y$: if we do, we go to $x$, and then we stay in $x$ forever.
Now, the complementary probability (the probability that you get from your starting vertex $u$ to $x$ within $k$ steps) can be computed by taking powers of the transition matrix of this Markov chain, as usual.
$endgroup$
To simplify the problem as much as possible, you can combine all vertices in $U$ into a single vertex $x$. Each edge from $v in Y$ to a vertex in $U$ becomes an edge from $v$ to $x$; if you get parallel edges this way, you should either keep them both, or else combine them by adding the weights.
Next, turn the random walk into an asymmetric Markov chain by making $x$ an absorbing state. This will make it easy to tell when we've left $Y$: if we do, we go to $x$, and then we stay in $x$ forever.
Now, the complementary probability (the probability that you get from your starting vertex $u$ to $x$ within $k$ steps) can be computed by taking powers of the transition matrix of this Markov chain, as usual.
answered Dec 1 '18 at 18:44
Misha LavrovMisha Lavrov
44.7k556107
44.7k556107
add a comment |
add a comment |
Thanks for contributing an answer to Mathematics Stack Exchange!
- Please be sure to answer the question. Provide details and share your research!
But avoid …
- Asking for help, clarification, or responding to other answers.
- Making statements based on opinion; back them up with references or personal experience.
Use MathJax to format equations. MathJax reference.
To learn more, see our tips on writing great answers.
Sign up or log in
StackExchange.ready(function () {
StackExchange.helpers.onClickDraftSave('#login-link');
});
Sign up using Google
Sign up using Facebook
Sign up using Email and Password
Post as a guest
Required, but never shown
StackExchange.ready(
function () {
StackExchange.openid.initPostLogin('.new-post-login', 'https%3a%2f%2fmath.stackexchange.com%2fquestions%2f3021633%2fprobability-that-a-random-walk-does-not-leave-a-subgraph-after-k-steps%23new-answer', 'question_page');
}
);
Post as a guest
Required, but never shown
Sign up or log in
StackExchange.ready(function () {
StackExchange.helpers.onClickDraftSave('#login-link');
});
Sign up using Google
Sign up using Facebook
Sign up using Email and Password
Post as a guest
Required, but never shown
Sign up or log in
StackExchange.ready(function () {
StackExchange.helpers.onClickDraftSave('#login-link');
});
Sign up using Google
Sign up using Facebook
Sign up using Email and Password
Post as a guest
Required, but never shown
Sign up or log in
StackExchange.ready(function () {
StackExchange.helpers.onClickDraftSave('#login-link');
});
Sign up using Google
Sign up using Facebook
Sign up using Email and Password
Sign up using Google
Sign up using Facebook
Sign up using Email and Password
Post as a guest
Required, but never shown
Required, but never shown
Required, but never shown
Required, but never shown
Required, but never shown
Required, but never shown
Required, but never shown
Required, but never shown
Required, but never shown
s I3nxBhHlEj,S,nYGHMaUy9mqAT,PRVElOq 38 WNItd 7 pm t9Tz D9uX5 2 n,Zcm0iSNh,6wQCTjZ qjIQNJh0ow,zm4gU