Expectation in spectral sparsification algorithms
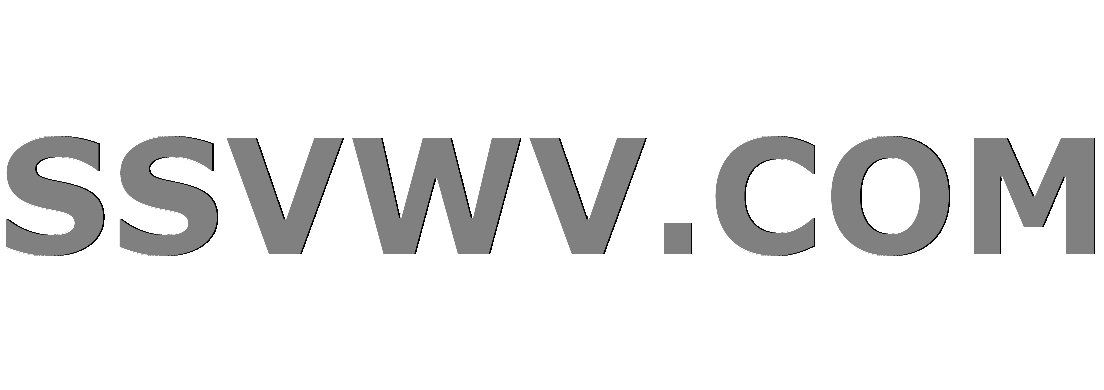
Multi tool use
$begingroup$
I am new to random matrices. I am studying the (Sampling) sparsification algorithms done by Daniel Spielman, Teng, Srivastava.
They used the concept of graph sampling to obtain a good spectral sparsifier $H $ for every graph $G $.
The sampling procedure involves assigning a probabilty $p_{u,v} $ to each edge $(u,v)$ in $G$ and then selecting edge $(u,v)$ to be in graph $H $ with probability $p_{u,v} $. When edge $(u,v) $ is chosen to be in $H $, we multiply its weight by $1/p_{u,v} $.
Apparently this procedure guarantees that the expectation of the Laplacian of $H$ is
$E (L_{H})= L_{G}$. Now I am not fully understanding how they figured out the expectation.please explain
probability algorithms random-matrices spectral-graph-theory algorithmic-randomness
$endgroup$
add a comment |
$begingroup$
I am new to random matrices. I am studying the (Sampling) sparsification algorithms done by Daniel Spielman, Teng, Srivastava.
They used the concept of graph sampling to obtain a good spectral sparsifier $H $ for every graph $G $.
The sampling procedure involves assigning a probabilty $p_{u,v} $ to each edge $(u,v)$ in $G$ and then selecting edge $(u,v)$ to be in graph $H $ with probability $p_{u,v} $. When edge $(u,v) $ is chosen to be in $H $, we multiply its weight by $1/p_{u,v} $.
Apparently this procedure guarantees that the expectation of the Laplacian of $H$ is
$E (L_{H})= L_{G}$. Now I am not fully understanding how they figured out the expectation.please explain
probability algorithms random-matrices spectral-graph-theory algorithmic-randomness
$endgroup$
add a comment |
$begingroup$
I am new to random matrices. I am studying the (Sampling) sparsification algorithms done by Daniel Spielman, Teng, Srivastava.
They used the concept of graph sampling to obtain a good spectral sparsifier $H $ for every graph $G $.
The sampling procedure involves assigning a probabilty $p_{u,v} $ to each edge $(u,v)$ in $G$ and then selecting edge $(u,v)$ to be in graph $H $ with probability $p_{u,v} $. When edge $(u,v) $ is chosen to be in $H $, we multiply its weight by $1/p_{u,v} $.
Apparently this procedure guarantees that the expectation of the Laplacian of $H$ is
$E (L_{H})= L_{G}$. Now I am not fully understanding how they figured out the expectation.please explain
probability algorithms random-matrices spectral-graph-theory algorithmic-randomness
$endgroup$
I am new to random matrices. I am studying the (Sampling) sparsification algorithms done by Daniel Spielman, Teng, Srivastava.
They used the concept of graph sampling to obtain a good spectral sparsifier $H $ for every graph $G $.
The sampling procedure involves assigning a probabilty $p_{u,v} $ to each edge $(u,v)$ in $G$ and then selecting edge $(u,v)$ to be in graph $H $ with probability $p_{u,v} $. When edge $(u,v) $ is chosen to be in $H $, we multiply its weight by $1/p_{u,v} $.
Apparently this procedure guarantees that the expectation of the Laplacian of $H$ is
$E (L_{H})= L_{G}$. Now I am not fully understanding how they figured out the expectation.please explain
probability algorithms random-matrices spectral-graph-theory algorithmic-randomness
probability algorithms random-matrices spectral-graph-theory algorithmic-randomness
edited Dec 10 '18 at 13:11
Sal
asked Dec 10 '18 at 10:41
SalSal
14010
14010
add a comment |
add a comment |
1 Answer
1
active
oldest
votes
$begingroup$
To figure out the correct weights $tilde{w}_e$ of the randomly sampled graph, it is useful to write the Laplacian of a weighted graph $G$ as the sum of outer products
$$L_G = sum_{e in E} w_e b_e b_e^T,$$
where
$w_e$ is the weight of the edge $e$ and $b_e$ is the vector such that
$$b_e(w) =
begin{cases}
1 & w = mathrm{head}(e)\
-1 & w = mathrm{tail}(e)\
0 & text{otherwise},
end{cases}$$
after some arbitrary orientation of the edge $e$.
Now if we sample each edge $e$ with probability $p_e$, we are left with a graph $tilde{G}$ whose Laplacian is
$$L_tilde{G} = sum_{e in E} I_e tilde{w}_e b_e b_e^T,$$
where $I_e sim textsf{Bern}(p_e)$ is an indicator random variable for whether or not $e$ was chosen. To make the expectation line up, we just need $mathbb{E}[I_e tilde{w}_e] = w_e$. In other words, we want $tilde{w}_e = frac{w_e}{mathbb{E}[I_e]} = frac{w_e}{p_e}$.
$endgroup$
add a comment |
Your Answer
StackExchange.ifUsing("editor", function () {
return StackExchange.using("mathjaxEditing", function () {
StackExchange.MarkdownEditor.creationCallbacks.add(function (editor, postfix) {
StackExchange.mathjaxEditing.prepareWmdForMathJax(editor, postfix, [["$", "$"], ["\\(","\\)"]]);
});
});
}, "mathjax-editing");
StackExchange.ready(function() {
var channelOptions = {
tags: "".split(" "),
id: "69"
};
initTagRenderer("".split(" "), "".split(" "), channelOptions);
StackExchange.using("externalEditor", function() {
// Have to fire editor after snippets, if snippets enabled
if (StackExchange.settings.snippets.snippetsEnabled) {
StackExchange.using("snippets", function() {
createEditor();
});
}
else {
createEditor();
}
});
function createEditor() {
StackExchange.prepareEditor({
heartbeatType: 'answer',
autoActivateHeartbeat: false,
convertImagesToLinks: true,
noModals: true,
showLowRepImageUploadWarning: true,
reputationToPostImages: 10,
bindNavPrevention: true,
postfix: "",
imageUploader: {
brandingHtml: "Powered by u003ca class="icon-imgur-white" href="https://imgur.com/"u003eu003c/au003e",
contentPolicyHtml: "User contributions licensed under u003ca href="https://creativecommons.org/licenses/by-sa/3.0/"u003ecc by-sa 3.0 with attribution requiredu003c/au003e u003ca href="https://stackoverflow.com/legal/content-policy"u003e(content policy)u003c/au003e",
allowUrls: true
},
noCode: true, onDemand: true,
discardSelector: ".discard-answer"
,immediatelyShowMarkdownHelp:true
});
}
});
Sign up or log in
StackExchange.ready(function () {
StackExchange.helpers.onClickDraftSave('#login-link');
});
Sign up using Google
Sign up using Facebook
Sign up using Email and Password
Post as a guest
Required, but never shown
StackExchange.ready(
function () {
StackExchange.openid.initPostLogin('.new-post-login', 'https%3a%2f%2fmath.stackexchange.com%2fquestions%2f3033760%2fexpectation-in-spectral-sparsification-algorithms%23new-answer', 'question_page');
}
);
Post as a guest
Required, but never shown
1 Answer
1
active
oldest
votes
1 Answer
1
active
oldest
votes
active
oldest
votes
active
oldest
votes
$begingroup$
To figure out the correct weights $tilde{w}_e$ of the randomly sampled graph, it is useful to write the Laplacian of a weighted graph $G$ as the sum of outer products
$$L_G = sum_{e in E} w_e b_e b_e^T,$$
where
$w_e$ is the weight of the edge $e$ and $b_e$ is the vector such that
$$b_e(w) =
begin{cases}
1 & w = mathrm{head}(e)\
-1 & w = mathrm{tail}(e)\
0 & text{otherwise},
end{cases}$$
after some arbitrary orientation of the edge $e$.
Now if we sample each edge $e$ with probability $p_e$, we are left with a graph $tilde{G}$ whose Laplacian is
$$L_tilde{G} = sum_{e in E} I_e tilde{w}_e b_e b_e^T,$$
where $I_e sim textsf{Bern}(p_e)$ is an indicator random variable for whether or not $e$ was chosen. To make the expectation line up, we just need $mathbb{E}[I_e tilde{w}_e] = w_e$. In other words, we want $tilde{w}_e = frac{w_e}{mathbb{E}[I_e]} = frac{w_e}{p_e}$.
$endgroup$
add a comment |
$begingroup$
To figure out the correct weights $tilde{w}_e$ of the randomly sampled graph, it is useful to write the Laplacian of a weighted graph $G$ as the sum of outer products
$$L_G = sum_{e in E} w_e b_e b_e^T,$$
where
$w_e$ is the weight of the edge $e$ and $b_e$ is the vector such that
$$b_e(w) =
begin{cases}
1 & w = mathrm{head}(e)\
-1 & w = mathrm{tail}(e)\
0 & text{otherwise},
end{cases}$$
after some arbitrary orientation of the edge $e$.
Now if we sample each edge $e$ with probability $p_e$, we are left with a graph $tilde{G}$ whose Laplacian is
$$L_tilde{G} = sum_{e in E} I_e tilde{w}_e b_e b_e^T,$$
where $I_e sim textsf{Bern}(p_e)$ is an indicator random variable for whether or not $e$ was chosen. To make the expectation line up, we just need $mathbb{E}[I_e tilde{w}_e] = w_e$. In other words, we want $tilde{w}_e = frac{w_e}{mathbb{E}[I_e]} = frac{w_e}{p_e}$.
$endgroup$
add a comment |
$begingroup$
To figure out the correct weights $tilde{w}_e$ of the randomly sampled graph, it is useful to write the Laplacian of a weighted graph $G$ as the sum of outer products
$$L_G = sum_{e in E} w_e b_e b_e^T,$$
where
$w_e$ is the weight of the edge $e$ and $b_e$ is the vector such that
$$b_e(w) =
begin{cases}
1 & w = mathrm{head}(e)\
-1 & w = mathrm{tail}(e)\
0 & text{otherwise},
end{cases}$$
after some arbitrary orientation of the edge $e$.
Now if we sample each edge $e$ with probability $p_e$, we are left with a graph $tilde{G}$ whose Laplacian is
$$L_tilde{G} = sum_{e in E} I_e tilde{w}_e b_e b_e^T,$$
where $I_e sim textsf{Bern}(p_e)$ is an indicator random variable for whether or not $e$ was chosen. To make the expectation line up, we just need $mathbb{E}[I_e tilde{w}_e] = w_e$. In other words, we want $tilde{w}_e = frac{w_e}{mathbb{E}[I_e]} = frac{w_e}{p_e}$.
$endgroup$
To figure out the correct weights $tilde{w}_e$ of the randomly sampled graph, it is useful to write the Laplacian of a weighted graph $G$ as the sum of outer products
$$L_G = sum_{e in E} w_e b_e b_e^T,$$
where
$w_e$ is the weight of the edge $e$ and $b_e$ is the vector such that
$$b_e(w) =
begin{cases}
1 & w = mathrm{head}(e)\
-1 & w = mathrm{tail}(e)\
0 & text{otherwise},
end{cases}$$
after some arbitrary orientation of the edge $e$.
Now if we sample each edge $e$ with probability $p_e$, we are left with a graph $tilde{G}$ whose Laplacian is
$$L_tilde{G} = sum_{e in E} I_e tilde{w}_e b_e b_e^T,$$
where $I_e sim textsf{Bern}(p_e)$ is an indicator random variable for whether or not $e$ was chosen. To make the expectation line up, we just need $mathbb{E}[I_e tilde{w}_e] = w_e$. In other words, we want $tilde{w}_e = frac{w_e}{mathbb{E}[I_e]} = frac{w_e}{p_e}$.
answered Dec 11 '18 at 0:58
Zach LangleyZach Langley
9731019
9731019
add a comment |
add a comment |
Thanks for contributing an answer to Mathematics Stack Exchange!
- Please be sure to answer the question. Provide details and share your research!
But avoid …
- Asking for help, clarification, or responding to other answers.
- Making statements based on opinion; back them up with references or personal experience.
Use MathJax to format equations. MathJax reference.
To learn more, see our tips on writing great answers.
Sign up or log in
StackExchange.ready(function () {
StackExchange.helpers.onClickDraftSave('#login-link');
});
Sign up using Google
Sign up using Facebook
Sign up using Email and Password
Post as a guest
Required, but never shown
StackExchange.ready(
function () {
StackExchange.openid.initPostLogin('.new-post-login', 'https%3a%2f%2fmath.stackexchange.com%2fquestions%2f3033760%2fexpectation-in-spectral-sparsification-algorithms%23new-answer', 'question_page');
}
);
Post as a guest
Required, but never shown
Sign up or log in
StackExchange.ready(function () {
StackExchange.helpers.onClickDraftSave('#login-link');
});
Sign up using Google
Sign up using Facebook
Sign up using Email and Password
Post as a guest
Required, but never shown
Sign up or log in
StackExchange.ready(function () {
StackExchange.helpers.onClickDraftSave('#login-link');
});
Sign up using Google
Sign up using Facebook
Sign up using Email and Password
Post as a guest
Required, but never shown
Sign up or log in
StackExchange.ready(function () {
StackExchange.helpers.onClickDraftSave('#login-link');
});
Sign up using Google
Sign up using Facebook
Sign up using Email and Password
Sign up using Google
Sign up using Facebook
Sign up using Email and Password
Post as a guest
Required, but never shown
Required, but never shown
Required, but never shown
Required, but never shown
Required, but never shown
Required, but never shown
Required, but never shown
Required, but never shown
Required, but never shown
nSg5pwMfwY,xvWxCdHzawib