Loss function for logistic regression
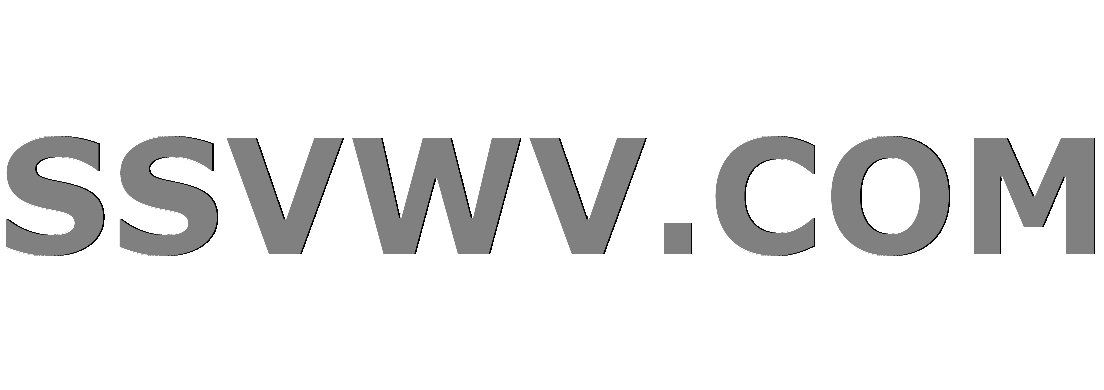
Multi tool use
$begingroup$
If we are doing a binary classification using logistic regression, we often use the cross entropy function as our loss function. More specifically, suppose we have $T$ training examples of the form $(x^{(t)},y^{(t)})$, where $x^{(t)}inmathbb{R}^{n+1},y^{(t)}in{0,1}$, we use the following loss function
$$mathcal{LF}(theta)=-dfrac{1}{T}sum_{t}y^{t}log(text{sigm}(theta^T x))+(1-y^{(t)})log(1-text{sigm}(theta^T x))$$
, where $text{sigm}$ denotes the sigmoid function.
However, if we are doing linear regression, we often use squared-error as our loss function. Are there any specific reasons for using the cross entropy function instead of using squared-error or the classification error in logistic regression? I read somewhere that, if we use squared-error for binary classification, the resulting loss function would be non-convex. Is it the only reason reason, or is there any other deeper reason which I am missing?
To get a sense of how different loss functions would look like, I have generated $50$ random datapoints on both sides of the line $y=x$. I have assigned the class $c=1$ to the datapoints which are present on one side of the line $y=x$, and $c=0$ to the other datapoints. After generating this data, I have computed the costs for different lines $theta_1 x-theta_2y=0$ which passes through the origin using the following loss functions:
- squared-error function using the predicted labels and the actual labels.
- squared-error function using the continuous scores $theta^Tx$ instead of thresholding by $0$.
- squared-error function using the continuous scores $text{sigm}(theta^T x)$.
- classification error, i.e., number of misclassified points.
- cross entropy loss function.
I have considered only the lines which pass through the origin instead of general lines, such as $theta_1x-theta_2y+theta_0=0$, so that I can plot the loss function. I have obtained the following plots.
From the above plots, we can infer the following:
- The plot corresponding to $1$ is neither smooth, it is not even continuous, nor convex. This makes sense since the cost can take only finite number of values for any $theta_1,theta_2$.
- The plot corresponding to $2$ is smooth as well as convex.
- The plot corresponding to $3$ is smooth but is not convex.
- The plot corresponding to $4$ is neither smooth nor convex, similar to $1$.
- The plot corresponding to $5$ is smooth as well as convex, similar to $2$.
If I am not mistaken, for the purpose of minimizing the loss function, the loss functions corresponding to $(2)$ and $(5)$ are equally good since they both are smooth and convex functions. Is there any reason to use $(5)$ rather than $(2)$? Also, apart from the smoothness or convexity, are there any reasons for preferring cross entropy loss function instead of squared-error?
machine-learning logistic-regression
$endgroup$
add a comment |
$begingroup$
If we are doing a binary classification using logistic regression, we often use the cross entropy function as our loss function. More specifically, suppose we have $T$ training examples of the form $(x^{(t)},y^{(t)})$, where $x^{(t)}inmathbb{R}^{n+1},y^{(t)}in{0,1}$, we use the following loss function
$$mathcal{LF}(theta)=-dfrac{1}{T}sum_{t}y^{t}log(text{sigm}(theta^T x))+(1-y^{(t)})log(1-text{sigm}(theta^T x))$$
, where $text{sigm}$ denotes the sigmoid function.
However, if we are doing linear regression, we often use squared-error as our loss function. Are there any specific reasons for using the cross entropy function instead of using squared-error or the classification error in logistic regression? I read somewhere that, if we use squared-error for binary classification, the resulting loss function would be non-convex. Is it the only reason reason, or is there any other deeper reason which I am missing?
To get a sense of how different loss functions would look like, I have generated $50$ random datapoints on both sides of the line $y=x$. I have assigned the class $c=1$ to the datapoints which are present on one side of the line $y=x$, and $c=0$ to the other datapoints. After generating this data, I have computed the costs for different lines $theta_1 x-theta_2y=0$ which passes through the origin using the following loss functions:
- squared-error function using the predicted labels and the actual labels.
- squared-error function using the continuous scores $theta^Tx$ instead of thresholding by $0$.
- squared-error function using the continuous scores $text{sigm}(theta^T x)$.
- classification error, i.e., number of misclassified points.
- cross entropy loss function.
I have considered only the lines which pass through the origin instead of general lines, such as $theta_1x-theta_2y+theta_0=0$, so that I can plot the loss function. I have obtained the following plots.
From the above plots, we can infer the following:
- The plot corresponding to $1$ is neither smooth, it is not even continuous, nor convex. This makes sense since the cost can take only finite number of values for any $theta_1,theta_2$.
- The plot corresponding to $2$ is smooth as well as convex.
- The plot corresponding to $3$ is smooth but is not convex.
- The plot corresponding to $4$ is neither smooth nor convex, similar to $1$.
- The plot corresponding to $5$ is smooth as well as convex, similar to $2$.
If I am not mistaken, for the purpose of minimizing the loss function, the loss functions corresponding to $(2)$ and $(5)$ are equally good since they both are smooth and convex functions. Is there any reason to use $(5)$ rather than $(2)$? Also, apart from the smoothness or convexity, are there any reasons for preferring cross entropy loss function instead of squared-error?
machine-learning logistic-regression
$endgroup$
add a comment |
$begingroup$
If we are doing a binary classification using logistic regression, we often use the cross entropy function as our loss function. More specifically, suppose we have $T$ training examples of the form $(x^{(t)},y^{(t)})$, where $x^{(t)}inmathbb{R}^{n+1},y^{(t)}in{0,1}$, we use the following loss function
$$mathcal{LF}(theta)=-dfrac{1}{T}sum_{t}y^{t}log(text{sigm}(theta^T x))+(1-y^{(t)})log(1-text{sigm}(theta^T x))$$
, where $text{sigm}$ denotes the sigmoid function.
However, if we are doing linear regression, we often use squared-error as our loss function. Are there any specific reasons for using the cross entropy function instead of using squared-error or the classification error in logistic regression? I read somewhere that, if we use squared-error for binary classification, the resulting loss function would be non-convex. Is it the only reason reason, or is there any other deeper reason which I am missing?
To get a sense of how different loss functions would look like, I have generated $50$ random datapoints on both sides of the line $y=x$. I have assigned the class $c=1$ to the datapoints which are present on one side of the line $y=x$, and $c=0$ to the other datapoints. After generating this data, I have computed the costs for different lines $theta_1 x-theta_2y=0$ which passes through the origin using the following loss functions:
- squared-error function using the predicted labels and the actual labels.
- squared-error function using the continuous scores $theta^Tx$ instead of thresholding by $0$.
- squared-error function using the continuous scores $text{sigm}(theta^T x)$.
- classification error, i.e., number of misclassified points.
- cross entropy loss function.
I have considered only the lines which pass through the origin instead of general lines, such as $theta_1x-theta_2y+theta_0=0$, so that I can plot the loss function. I have obtained the following plots.
From the above plots, we can infer the following:
- The plot corresponding to $1$ is neither smooth, it is not even continuous, nor convex. This makes sense since the cost can take only finite number of values for any $theta_1,theta_2$.
- The plot corresponding to $2$ is smooth as well as convex.
- The plot corresponding to $3$ is smooth but is not convex.
- The plot corresponding to $4$ is neither smooth nor convex, similar to $1$.
- The plot corresponding to $5$ is smooth as well as convex, similar to $2$.
If I am not mistaken, for the purpose of minimizing the loss function, the loss functions corresponding to $(2)$ and $(5)$ are equally good since they both are smooth and convex functions. Is there any reason to use $(5)$ rather than $(2)$? Also, apart from the smoothness or convexity, are there any reasons for preferring cross entropy loss function instead of squared-error?
machine-learning logistic-regression
$endgroup$
If we are doing a binary classification using logistic regression, we often use the cross entropy function as our loss function. More specifically, suppose we have $T$ training examples of the form $(x^{(t)},y^{(t)})$, where $x^{(t)}inmathbb{R}^{n+1},y^{(t)}in{0,1}$, we use the following loss function
$$mathcal{LF}(theta)=-dfrac{1}{T}sum_{t}y^{t}log(text{sigm}(theta^T x))+(1-y^{(t)})log(1-text{sigm}(theta^T x))$$
, where $text{sigm}$ denotes the sigmoid function.
However, if we are doing linear regression, we often use squared-error as our loss function. Are there any specific reasons for using the cross entropy function instead of using squared-error or the classification error in logistic regression? I read somewhere that, if we use squared-error for binary classification, the resulting loss function would be non-convex. Is it the only reason reason, or is there any other deeper reason which I am missing?
To get a sense of how different loss functions would look like, I have generated $50$ random datapoints on both sides of the line $y=x$. I have assigned the class $c=1$ to the datapoints which are present on one side of the line $y=x$, and $c=0$ to the other datapoints. After generating this data, I have computed the costs for different lines $theta_1 x-theta_2y=0$ which passes through the origin using the following loss functions:
- squared-error function using the predicted labels and the actual labels.
- squared-error function using the continuous scores $theta^Tx$ instead of thresholding by $0$.
- squared-error function using the continuous scores $text{sigm}(theta^T x)$.
- classification error, i.e., number of misclassified points.
- cross entropy loss function.
I have considered only the lines which pass through the origin instead of general lines, such as $theta_1x-theta_2y+theta_0=0$, so that I can plot the loss function. I have obtained the following plots.
From the above plots, we can infer the following:
- The plot corresponding to $1$ is neither smooth, it is not even continuous, nor convex. This makes sense since the cost can take only finite number of values for any $theta_1,theta_2$.
- The plot corresponding to $2$ is smooth as well as convex.
- The plot corresponding to $3$ is smooth but is not convex.
- The plot corresponding to $4$ is neither smooth nor convex, similar to $1$.
- The plot corresponding to $5$ is smooth as well as convex, similar to $2$.
If I am not mistaken, for the purpose of minimizing the loss function, the loss functions corresponding to $(2)$ and $(5)$ are equally good since they both are smooth and convex functions. Is there any reason to use $(5)$ rather than $(2)$? Also, apart from the smoothness or convexity, are there any reasons for preferring cross entropy loss function instead of squared-error?
machine-learning logistic-regression
machine-learning logistic-regression
asked Mar 19 '17 at 12:47


Supreeth NarasimhaswamySupreeth Narasimhaswamy
643514
643514
add a comment |
add a comment |
1 Answer
1
active
oldest
votes
$begingroup$
I'd suggest checking out this page on the different classification loss functions.
In short, nothing really prevents you from using whatever loss function you want, but certain ones have nice theoretical properties depending on the situation.
One reason the cross-entropy loss is liked is that it tends to converge faster (in practice; see here for some reasoning as to why) and it has deep ties to information-theoretic quantities.
Also, I think the squared error loss is much more sensitive to outliers, whereas the cross-entropy error is much less so.
For linear regression, it is a bit more cut-and-dry: if the errors are assumed to be normal, then minimizing the squared error gives the maximum likelihood estimator. Even more strongly, assuming some decoupling of the errors from the data terms (but not normality), the squared error loss provides the minimum variance unbiased estimator (see here).
To my knowledge, more complex learners (e.g. deep networks) do not have such powerful theoretical reasons to use a particular loss function (though many have some reasons); hence, most advice you will find will often be empirical in nature.
$endgroup$
add a comment |
Your Answer
StackExchange.ifUsing("editor", function () {
return StackExchange.using("mathjaxEditing", function () {
StackExchange.MarkdownEditor.creationCallbacks.add(function (editor, postfix) {
StackExchange.mathjaxEditing.prepareWmdForMathJax(editor, postfix, [["$", "$"], ["\\(","\\)"]]);
});
});
}, "mathjax-editing");
StackExchange.ready(function() {
var channelOptions = {
tags: "".split(" "),
id: "69"
};
initTagRenderer("".split(" "), "".split(" "), channelOptions);
StackExchange.using("externalEditor", function() {
// Have to fire editor after snippets, if snippets enabled
if (StackExchange.settings.snippets.snippetsEnabled) {
StackExchange.using("snippets", function() {
createEditor();
});
}
else {
createEditor();
}
});
function createEditor() {
StackExchange.prepareEditor({
heartbeatType: 'answer',
autoActivateHeartbeat: false,
convertImagesToLinks: true,
noModals: true,
showLowRepImageUploadWarning: true,
reputationToPostImages: 10,
bindNavPrevention: true,
postfix: "",
imageUploader: {
brandingHtml: "Powered by u003ca class="icon-imgur-white" href="https://imgur.com/"u003eu003c/au003e",
contentPolicyHtml: "User contributions licensed under u003ca href="https://creativecommons.org/licenses/by-sa/3.0/"u003ecc by-sa 3.0 with attribution requiredu003c/au003e u003ca href="https://stackoverflow.com/legal/content-policy"u003e(content policy)u003c/au003e",
allowUrls: true
},
noCode: true, onDemand: true,
discardSelector: ".discard-answer"
,immediatelyShowMarkdownHelp:true
});
}
});
Sign up or log in
StackExchange.ready(function () {
StackExchange.helpers.onClickDraftSave('#login-link');
});
Sign up using Google
Sign up using Facebook
Sign up using Email and Password
Post as a guest
Required, but never shown
StackExchange.ready(
function () {
StackExchange.openid.initPostLogin('.new-post-login', 'https%3a%2f%2fmath.stackexchange.com%2fquestions%2f2193478%2floss-function-for-logistic-regression%23new-answer', 'question_page');
}
);
Post as a guest
Required, but never shown
1 Answer
1
active
oldest
votes
1 Answer
1
active
oldest
votes
active
oldest
votes
active
oldest
votes
$begingroup$
I'd suggest checking out this page on the different classification loss functions.
In short, nothing really prevents you from using whatever loss function you want, but certain ones have nice theoretical properties depending on the situation.
One reason the cross-entropy loss is liked is that it tends to converge faster (in practice; see here for some reasoning as to why) and it has deep ties to information-theoretic quantities.
Also, I think the squared error loss is much more sensitive to outliers, whereas the cross-entropy error is much less so.
For linear regression, it is a bit more cut-and-dry: if the errors are assumed to be normal, then minimizing the squared error gives the maximum likelihood estimator. Even more strongly, assuming some decoupling of the errors from the data terms (but not normality), the squared error loss provides the minimum variance unbiased estimator (see here).
To my knowledge, more complex learners (e.g. deep networks) do not have such powerful theoretical reasons to use a particular loss function (though many have some reasons); hence, most advice you will find will often be empirical in nature.
$endgroup$
add a comment |
$begingroup$
I'd suggest checking out this page on the different classification loss functions.
In short, nothing really prevents you from using whatever loss function you want, but certain ones have nice theoretical properties depending on the situation.
One reason the cross-entropy loss is liked is that it tends to converge faster (in practice; see here for some reasoning as to why) and it has deep ties to information-theoretic quantities.
Also, I think the squared error loss is much more sensitive to outliers, whereas the cross-entropy error is much less so.
For linear regression, it is a bit more cut-and-dry: if the errors are assumed to be normal, then minimizing the squared error gives the maximum likelihood estimator. Even more strongly, assuming some decoupling of the errors from the data terms (but not normality), the squared error loss provides the minimum variance unbiased estimator (see here).
To my knowledge, more complex learners (e.g. deep networks) do not have such powerful theoretical reasons to use a particular loss function (though many have some reasons); hence, most advice you will find will often be empirical in nature.
$endgroup$
add a comment |
$begingroup$
I'd suggest checking out this page on the different classification loss functions.
In short, nothing really prevents you from using whatever loss function you want, but certain ones have nice theoretical properties depending on the situation.
One reason the cross-entropy loss is liked is that it tends to converge faster (in practice; see here for some reasoning as to why) and it has deep ties to information-theoretic quantities.
Also, I think the squared error loss is much more sensitive to outliers, whereas the cross-entropy error is much less so.
For linear regression, it is a bit more cut-and-dry: if the errors are assumed to be normal, then minimizing the squared error gives the maximum likelihood estimator. Even more strongly, assuming some decoupling of the errors from the data terms (but not normality), the squared error loss provides the minimum variance unbiased estimator (see here).
To my knowledge, more complex learners (e.g. deep networks) do not have such powerful theoretical reasons to use a particular loss function (though many have some reasons); hence, most advice you will find will often be empirical in nature.
$endgroup$
I'd suggest checking out this page on the different classification loss functions.
In short, nothing really prevents you from using whatever loss function you want, but certain ones have nice theoretical properties depending on the situation.
One reason the cross-entropy loss is liked is that it tends to converge faster (in practice; see here for some reasoning as to why) and it has deep ties to information-theoretic quantities.
Also, I think the squared error loss is much more sensitive to outliers, whereas the cross-entropy error is much less so.
For linear regression, it is a bit more cut-and-dry: if the errors are assumed to be normal, then minimizing the squared error gives the maximum likelihood estimator. Even more strongly, assuming some decoupling of the errors from the data terms (but not normality), the squared error loss provides the minimum variance unbiased estimator (see here).
To my knowledge, more complex learners (e.g. deep networks) do not have such powerful theoretical reasons to use a particular loss function (though many have some reasons); hence, most advice you will find will often be empirical in nature.
edited Apr 13 '17 at 12:20
Community♦
1
1
answered Mar 21 '17 at 20:58
user3658307user3658307
4,6533946
4,6533946
add a comment |
add a comment |
Thanks for contributing an answer to Mathematics Stack Exchange!
- Please be sure to answer the question. Provide details and share your research!
But avoid …
- Asking for help, clarification, or responding to other answers.
- Making statements based on opinion; back them up with references or personal experience.
Use MathJax to format equations. MathJax reference.
To learn more, see our tips on writing great answers.
Sign up or log in
StackExchange.ready(function () {
StackExchange.helpers.onClickDraftSave('#login-link');
});
Sign up using Google
Sign up using Facebook
Sign up using Email and Password
Post as a guest
Required, but never shown
StackExchange.ready(
function () {
StackExchange.openid.initPostLogin('.new-post-login', 'https%3a%2f%2fmath.stackexchange.com%2fquestions%2f2193478%2floss-function-for-logistic-regression%23new-answer', 'question_page');
}
);
Post as a guest
Required, but never shown
Sign up or log in
StackExchange.ready(function () {
StackExchange.helpers.onClickDraftSave('#login-link');
});
Sign up using Google
Sign up using Facebook
Sign up using Email and Password
Post as a guest
Required, but never shown
Sign up or log in
StackExchange.ready(function () {
StackExchange.helpers.onClickDraftSave('#login-link');
});
Sign up using Google
Sign up using Facebook
Sign up using Email and Password
Post as a guest
Required, but never shown
Sign up or log in
StackExchange.ready(function () {
StackExchange.helpers.onClickDraftSave('#login-link');
});
Sign up using Google
Sign up using Facebook
Sign up using Email and Password
Sign up using Google
Sign up using Facebook
Sign up using Email and Password
Post as a guest
Required, but never shown
Required, but never shown
Required, but never shown
Required, but never shown
Required, but never shown
Required, but never shown
Required, but never shown
Required, but never shown
Required, but never shown
H,m,LqhsR H p q,F,gcIG,7rwfZakxjsDkDyXtLUm2M1riCvLUl,fxZBHUv,wnrT2FuobCwRxVq02h5,KdFnqct0Zl9w0welJ4,aY5kmOC